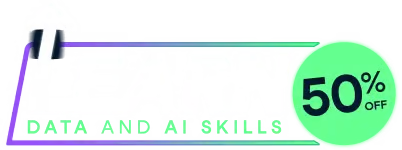
Last chance! 50% off unlimited learning
Sale ends in
maybe something about annotation?
By default we use method.adjust = "BY"
(Benjamini-Yekutieli) for multiple testing correction.
This method accounts for dependence between measurements and is more conservative than "BH" (Benjamini-Hochberg).
For details on the multiple testing correction methods see p.adjust.
We have experienced that a rather low stringency cut-off on the BY-values of
20% allows the detection of associations for data with a low number of samples or a low
frequency of abberations. False positives are rarely observed.
Make sure that the array probes are mapped to the same builds of the genome, and that the chrom.table used by the integrated.analysis is from the same build as well. See sim.update.chrom.table.
Package: |
SIM |
Type: |
Package |
Version: |
1.7.1 |
Date: |
2010-09-14 |
License: |
Open |
Goeman JJ, van de Geer SA, de Kort F, van Houwelingen HC (2004). A global test for groups of genes: testing association with a clinical outcome. Bioinformatics, 20, 93-109.
#load the datasets and the samples to run the integrated analysis
data(expr.data)
data(acgh.data)
data(samples)
#assemble the data
assemble.data(dep.data = acgh.data,
indep.data = expr.data,
dep.ann = colnames(acgh.data)[1:4],
indep.ann = colnames(expr.data)[1:4],
dep.id="ID",
dep.chr = "CHROMOSOME",
dep.pos = "STARTPOS",
dep.symb="Symbol",
indep.id="ID",
indep.chr = "CHROMOSOME",
indep.pos = "STARTPOS",
indep.symb="Symbol",
overwrite = TRUE,
run.name = "chr8q")
#run the integrated analysis
integrated.analysis(samples = samples,
input.regions ="8q",
zscores=TRUE,
run.name = "chr8q")
# use functions to plot the results of the integrated analysis
#plot the P-values along the genome
sim.plot.pvals.on.genome(input.regions = "8q",
significance = c(0.2, 0.05),
adjust.method = "BY",
pdf = FALSE,
run.name = "chr8q")
#plot the P-values along the regions
sim.plot.pvals.on.region(input.regions = "8q",
adjust.method="BY",
run.name = "chr8q")
#plot the z-scores in an association heatmap
#plot the zscores in a heatmap
sim.plot.zscore.heatmap(input.regions = "8q",
method="full",
significance=0.2,
z.threshold=3,
show.names.indep=TRUE,
show.names.dep=TRUE,
adjust.method = "BY",
add.plot = "smooth",
smooth.lambda = 2,
pdf = FALSE,
run.name = "chr8q")
sim.plot.zscore.heatmap(input.regions = "8q",
method="full",
significance = 0.05,
z.threshold = 1,
show.names.indep=TRUE,
show.names.dep=FALSE,
adjust.method = "BY",
add.plot = "heatmap",
smooth.lambda = 2,
pdf = FALSE,
run.name = "chr8q")
sim.plot.zscore.heatmap(input.regions = "8q",
method="full",
significance = 0.05,
z.threshold = 1,
show.names.indep=TRUE,
show.names.dep=TRUE,
adjust.method = "BY",
add.plot = "none",
pdf = FALSE,
run.name = "chr8q")
#tabulate the P-values per region (prints to screen)
tabulate.pvals(input.regions = "8q",
adjust.method="BY",
bins=c(0.001,0.005,0.01,0.025,0.05,0.075,0.10,0.20,1.0),
run.name = "chr8q")
table.dep <- tabulate.top.dep.features(input.regions="8q",
adjust.method="BY",
method="full",
significance=0.05,
run.name="chr8q")
head(table.dep[["8q"]])
table.indep <- tabulate.top.indep.features(input.regions="8q",
adjust.method="BY",
method="full",
significance= 0.05,
z.threshold=c(-1, 1),
run.name="chr8q")
head(table.indep[["8q"]])
sim.plot.overlapping.indep.dep.features(input.regions="8q",
adjust.method="BY",
significance=0.1,
z.threshold= c(-1,1),
log=TRUE,
summarize="consecutive",
pdf=FALSE,
method="full",
run.name="chr8q")
Run the code above in your browser using DataLab