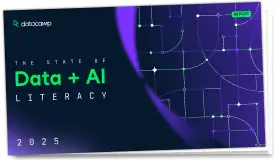
This algorithm is based on the interlacing property of eigenvalues. It starts with an initial solution given directly or provided by the greedy or dual-greedy approach. It uses a branch-and-bound strategy to calculate an optimal solution.
It is also possible to construct a completely new network, that means $nf=0$.
maxentropy(A,nf,ns,method="d",S.start=NULL,rtol=1e-6,mattest=TRUE,etol=0,verbose=FALSE)
"d"
=dual-greedy algorithm, "g"
=greedy algorithm, "dc"
=dual-greedy +
interchange algorithm, "gc"
=greedy + interchange algorithm,
"c"
=interchange algorithm + directly given initial solution. Otherwise
this algorithm has to be started with an directly given initial solution.TRUE
a tes for for symmetry and
positive definiteness of the matrix $A$ isperformed (default is
TRUE
).TRUE
some information is printed per
iteration (default is FALSE
).monet
containing the following elements:
Gebhardt, C.: Bayessche Methoden in der geostatistischen Versuchsplanung. PhD Thesis, Univ. Klagenfurt, Austria, 2003
O.P. Baume, A. Gebhardt, C. Gebhardt, G.B.M. Heuvelink and J. Pilz: Network optimization algorithms and scenarios in the context of automatic mapping. Computers & Geosciences 37 (2011) 3, 289-294
greedy
, dualgreedy
, interchange
x <- c(0.97900601,0.82658702,0.53105628,0.91420190,0.35304969,
0.14768239,0.58000004,0.60690101,0.36289026,0.82022147,
0.95290664,0.07928365,0.04833764,0.55631735,0.06427738,
0.31216689,0.43851418,0.34433556,0.77699357,0.84097327)
y <- c(0.36545512,0.72144122,0.95688671,0.25422154,0.48199229,
0.43874199,0.90166634,0.60898628,0.82634713,0.29670695,
0.86879093,0.45277452,0.09386800,0.04788365,0.20557817,
0.61149264,0.94643855,0.78219937,0.53946353,0.70946842)
A <- outer(x, x, "-")^2 + outer(y, y, "-")^2
A <- (2 - A)/10
diag(A) <- 0
diag(A) <- 1/20 + apply(A, 2, sum)
S.entrp<-c(0,7,0,9,0,11,0,13,14,0,0,0,0,0,0)
maxentropy(A,5,5,S.start=S.entrp)
maxentropy(A,5,5,method="g")
maxentropy(A,5,5)
Run the code above in your browser using DataLab