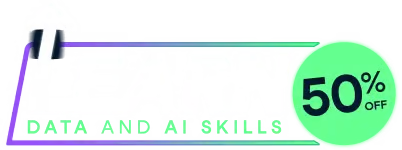
Last chance! 50% off unlimited learning
Sale ends in
plotLinearTrendTestDesign(x.var = "n", y.var = "power",
range.x.var = NULL, n = 12,
slope.over.sigma = switch(alternative, greater = 0.1, less = -0.1,
two.sided = ifelse(two.sided.direction == "greater", 0.1, -0.1)),
alpha = 0.05, power = 0.95, alternative = "two.sided",
two.sided.direction = "greater", approx = FALSE, round.up = FALSE,
n.max = 5000, tol = 1e-07, maxiter = 1000, plot.it = TRUE, add = FALSE,
n.points = ifelse(x.var == "n", diff(range.x.var) + 1, 50),
plot.col = "black", plot.lwd = 3 * par("cex"), plot.lty = 1,
digits = .Options$digits, ..., main = NULL, xlab = NULL, ylab = NULL,
type = "l")
"n"
(sample size; the default),
"slope.over.sigma"
(scaled minimal detectable slope), "power"
(power of the test), and"power"
(power of the test; the default),
"slope.over.sigma"
(scaled minimal detectable slope), and
"n"
(sample size).x.var
.
When x.var="n"
the default value is c(3,25)
. When
x.var="sl
n=12
. Missing (NA
), undefined (NaN
),
and infinite (Inf
, -Inf
) values are not allowed. This
argument is ignored alternative="greater"
or
alternative="two.s
alpha=0.05
.
This argument is ignored when x.var="alpha"
.power=0.95
. This argument is
ignored when x.var="power"
or y.var="power"
."two.sided"
(the default), "greater"
, and "less"
.alternative="two.sided"
.
When two.sided.direction="greater"
(the default), the scaled minimal
detectable sapprox=FALSE
.FALSE
.y.var="n"
, a positive integer greater than 2 indicating
the maximum sample size. The default value is n.max=5000
.uniroot
search algorithm.
The default value is tol=1e-7
.uniroot
function. The default
value is maxiter=1000
.add
) on the current graphics device. If plot.it=FALSE
, no plot
is produced, but a list of (x,y) values is returned (see VALUE). Tadd=TRUE
), or to create a plot from scratch
(add=FALSE
). The default value is add=FALSE
.
This argument is ignored if n.points
x-values evenly spaced between
range.x.var[1]
and range.x.var[2]
. The default value is
n.points=50
plot.col="black"
. See the
entry for col
in the help file for par
3*par("cex")
. See the entry for lwd
in the help file for par
for more information.plot.lty=1
. See the entry for lty
in the help file for par
for more information.options("digits")
.par
).plotlinearTrendTestDesign
invisibly returns a list with components
x.var
and y.var
, giving coordinates of the points that have
been or would have been plotted.linearTrendTestPower
,
linearTrendTestN
, and linearTrendTestScaledMds
for
information on how to compute the power, sample size, or scaled minimal detectable
slope for a t-test for linear trend.linearTrendTestPower
.linearTrendTestPower
, linearTrendTestN
,
linearTrendTestScaledMds
.# Look at the relationship between power and sample size for the t-test for
# liner trend, assuming a scaled slope of 0.1 and a 5% significance level:
dev.new()
plotLinearTrendTestDesign()
#==========
# Plot sample size vs. the scaled minimal detectable slope for various
# levels of power, using a 5% significance level:
dev.new()
plotLinearTrendTestDesign(x.var = "slope.over.sigma", y.var = "n",
ylim = c(0, 30), main = "")
plotLinearTrendTestDesign(x.var = "slope.over.sigma", y.var = "n",
power = 0.9, add = TRUE, plot.col = "red")
plotLinearTrendTestDesign(x.var = "slope.over.sigma", y.var = "n",
power = 0.8, add = TRUE, plot.col = "blue")
legend("topright", c("95%", "90%", "80%"), lty = 1, bty = "n",
lwd = 3 * par("cex"), col = c("black", "red", "blue"))
title(main = paste("Sample Size vs. Scaled Slope for t-Test for Linear Trend",
"with Alpha=0.05 and Various Powers", sep=""))
#==========
# Clean up
#---------
graphics.off()
Run the code above in your browser using DataLab