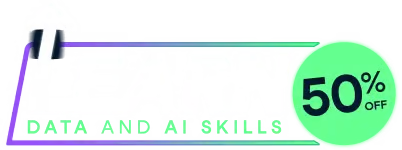
Last chance! 50% off unlimited learning
Sale ends in
to_factor(x, add.non.labelled = FALSE, drop.na = TRUE)to_fac(x, add.non.labelled = FALSE, drop.na = TRUE)
TRUE
, non-labelled values also
get value labels.TRUE
, all types of missing value codes are
converted into NA before x
is converted as factor. If
FALSE
, missing values will be left as their original codes.
See 'Examples' and
x
was a data frame.get_na
.to_value
to convert a factor into a numeric value and
to_label
to convert a value into a factor with labelled
factor levels.data(efc)
library(sjPlot)
# normal factor conversion, loses value attributes
efc$e42dep <- as.factor(efc$e42dep)
sjt.frq(efc$e42dep)
# factor conversion, which keeps value attributes
efc$e42dep <- to_factor(efc$e42dep)
sjt.frq(efc$e42dep)
data(efc)
# create parially labelled vector
x <- set_labels(efc$e42dep,
c(`1` = "independent",
`4` = "severe dependency",
`9` = "missing value"))
# only copy existing value labels
to_factor(x)
get_labels(to_factor(x), include.values = "p")
# also add labels to non-labelled values
to_factor(x, add.non.labelled = TRUE)
get_labels(to_factor(x, add.non.labelled = TRUE), include.values = "p")
# create labelled integer, with missing flag
x <- labelled(c(1, 2, 1, 3, 4, 1),
c(Male = 1, Female = 2, Refused = 3, "N/A" = 4),
c(FALSE, FALSE, TRUE, TRUE))
# to factor, with missing labels
to_factor(x, drop.na = FALSE)
# to factor, missings removed
to_factor(x, drop.na = TRUE)
Run the code above in your browser using DataLab