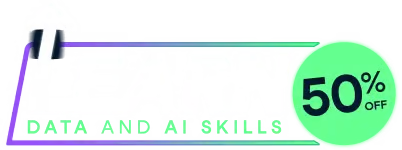
Last chance! 50% off unlimited learning
Sale ends in
DunnTest(x, ...)
## S3 method for class 'default':
DunnTest(x, g,
method = c("holm", "hochberg", "hommel", "bonferroni", "BH",
"BY", "fdr", "none"),
out.list = TRUE, ...)
## S3 method for class 'formula':
DunnTest(formula, data, subset, na.action,
method = c("holm", "hochberg", "hommel", "bonferroni", "BH",
"BY", "fdr", "none"),
out.list = TRUE, ...)
## S3 method for class 'DunnTest':
print(x, digits = getOption("digits"), ...)
x
. Ignored if x
is a
list.p.adjust
and this parameter is directly passed through.lhs ~ rhs
where lhs
gives the data values and rhs
the corresponding groups.model.frame
) containing the variables in the
formula formula
. By default the variables are taken from
environment(for
NA
s. Defaults to
getOption("na.action")
."DunnTest"
containing the following components:DunnTest
does the post hoc pairwise multiple comparisons procedure appropriate to follow the rejection of a Kruskal-Wallis test. The Kruskal-Wallis test, being a non-parametric analog of the one-way ANOVA, is an omnibus test of the null hypothesis that none of k groups stochastically dominate one another.
Dunn's test is constructed in part by summing jointly ranked data. The rank sum test, itself a non-parametric analog of the unpaired t-test, is possibly intuitive, but inappropriate as a post hoc pairwise test, because (1) it fails to retain the dependent ranking that produced the Kruskal-Wallis test statistic, and (2) it does not incorporate the pooled variance estimate implied by the null hypothesis of the Kruskal-Wallis test.
If x
is a list, its elements are taken as the samples to be
compared, and hence have to be numeric data vectors. In this case,
g
is ignored, and one can simply use DunnTest(x)
to perform the test. If the samples are not yet contained in a
list, use DunnTest(list(x, ...))
.
Otherwise, x
must be a numeric data vector, and g
must
be a vector or factor object of the same length as x
giving
the group for the corresponding elements of x
.kruskal.test
, wilcox.test
, p.adjust
## Hollander & Wolfe (1973), 116.
## Mucociliary efficiency from the rate of removal of dust in normal
## subjects, subjects with obstructive airway disease, and subjects
## with asbestosis.
x <- c(2.9, 3.0, 2.5, 2.6, 3.2) # normal subjects
y <- c(3.8, 2.7, 4.0, 2.4) # with obstructive airway disease
z <- c(2.8, 3.4, 3.7, 2.2, 2.0) # with asbestosis
DunnTest(list(x, y, z))
## Equivalently,
x <- c(x, y, z)
g <- factor(rep(1:3, c(5, 4, 5)),
labels = c("Normal subjects",
"Subjects with obstructive airway disease",
"Subjects with asbestosis"))
# do the kruskal.test first
kruskal.test(x, g)
# ...and the pairwise test afterwards
DunnTest(x, g)
## Formula interface.
require(graphics)
boxplot(Ozone ~ Month, data = airquality)
DunnTest(Ozone ~ Month, data = airquality)
Run the code above in your browser using DataLab