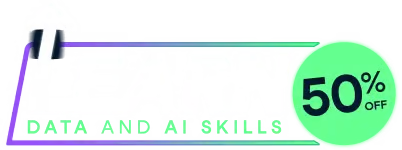
Last chance! 50% off unlimited learning
Sale ends in
serialCorrelationTest
is a generic function used to test for the
presence of lag-one serial correlation using either the rank
von Neumann ratio test, the normal approximation based on the Yule-Walker
estimate of lag-one correlation, or the normal approximation based on the
MLE of lag-one correlation. The function invokes particular
methods
which depend on the class
of the first
argument.
Currently, there is a default method and a method for objects of class "lm"
.
serialCorrelationTest(x, ...) # S3 method for default
serialCorrelationTest(x, test = "rank.von.Neumann",
alternative = "two.sided", conf.level = 0.95, ...)
# S3 method for lm
serialCorrelationTest(x, test = "rank.von.Neumann",
alternative = "two.sided", conf.level = 0.95, ...)
numeric vector of observations, a numeric univariate time series of
class "ts"
, or an object of class "lm"
. Undefined (NaN
) and
infinite (Inf
, -Inf
) values are not allowed for x
when x
is a numeric vector or time series, nor for the residuals
associated with x
when x
is an object of class "lm"
.
When test="AR1.mle"
, missing (NA
) values are allowed, otherwise
they are not allowed. When x
is a numeric vector of observations
or a numeric univariate time series of class "ts"
, it must contain at least
3 non-missing values. When x
is an object of class "lm"
, the
residuals must contain at least 3 non-missing values.
Note: when x
is an object of class "lm"
, the linear model
should have been fit using the argument na.action=na.exclude
in the
call to lm
in order to correctly deal with missing values.
character string indicating which test to use. The possible values are:
"rank.von.Neumann"
(rank von Neumann ratio test; the default),
"AR1.yw"
(z-test based on Yule-Walker lag-one estimate of correlation), and
"AR1.mle"
(z-test based on MLE of lag-one correlation).
character string indicating the kind of alternative hypothesis. The possible
values are "two.sided"
(the default), "greater"
, and "less"
.
numeric scalar between 0 and 1 indicating the confidence level associated with
the confidence interval for the population lag-one autocorrelation. The default
value is conf.level=0.95
.
optional arguments for possible future methods. Currently not used.
A list of class "htest"
containing the results of the hypothesis test.
See the help file for htest.object
for details.
Let serialCorrelationTest
tests the null hypothesis:
In the case when the argument x
is a linear model, the function
serialCorrelationTest
tests the null hypothesis (1) for the
residuals.
The three possible alternative hypotheses are the upper one-sided alternative
(alternative="greater"
):
alternative="less"
):
Testing the Null Hypothesis of No Lag-1 Autocorrelation
There are several possible methods for testing the null hypothesis (1) versus any
of the three alternatives (2)-(4). The function serialCorrelationTest
allows
you to use one of three possible tests:
The rank von Neuman ratio test.
The test based on the normal approximation for the distribution of the Yule-Walker estimate of lag-one correlation.
The test based on the normal approximation for the distribution of the maximum likelihood estimate (MLE) of lag-one correlation.
Each of these tests is described below.
Test Based on Yule-Walker Estimate (test="AR1.yw"
)
The Yule-Walker estimate of the lag-1 autocorrelation is given by:
Under the null hypothesis (1), the estimator of lag-1 correlation in Equation (5) is
approximately distributed as a normal (Gaussian) random variable with mean 0 and
variance given by:
Test Based on the MLE (test="AR1.mle"
)
The function serialCorrelationTest
the R function arima
to
compute the MLE of the lag-one autocorrelation and the estimated variance of this
estimator. As for the test based on the Yule-Walker estimate, the z-statistic is
computed as the estimated lag-one autocorrelation divided by the square root of the
estimated variance.
Test Based on Rank von Neumann Ratio (test="rank.von.Neumann"
)
The null distribution of the serial correlation coefficient may be badly affected
by departures from normality in the underlying process (Cox, 1966; Bartels, 1977).
It is therefore a good idea to consider using a nonparametric test for randomness if
the normality of the underlying process is in doubt (Bartels, 1982).
Wald and Wolfowitz (1943) introduced the rank serial correlation coefficient, which for lag-1 autocorrelation is simply the Yule-Walker estimate (Equation (5) above) with the actual observations replaced with their ranks.
von Neumann et al. (1941) introduced a test for randomness in the context of
testing for trend in the mean of a process. Their statistic is given by:
The rank version of the von Neumann ratio statistic is given by:
Bartels (1982) shows that asymptotically, the rank von Neumann ratio statistic is a linear transformation of the rank serial correlation coefficient, so any asymptotic results apply to both statistics.
For any fixed sample size
Determining the exact distribution of shape1=
shape2=
Note: The definition of the beta distribution assumes the
random variable ranges from 0 to 1. This definition can be generalized as follows.
Suppose the random variable
Bartels (1982) shows that asymptotically,
To test the null hypothesis (1) when test="rank.von.Neumann"
, the function
serialCorrelationTest
does the following:
When the sample size is between 3 and 10, the exact distribution of
When the sample size is between 11 and 100, the beta approximation to the
distribution of
When the sample size is larger than 100, the normal approximation to the
distribution of
When ties are present in the observations and midranks are used for the tied
observations, the distribution of the
When ties are present, the function serialCorrelationTest
issues a warning.
When the sample size is between 3 and 10, the p-value is computed based on
rounding up the computed value of
Computing a Confidence Interval for the Lag-1 Autocorrelation
The function serialCorrelationTest
computes an approximate
When test="AR1.yw"
or test="rank.von.Neumann"
, the Yule-Walker
estimate of lag-1 autocorrelation is used and the variance of the estimated
lag-1 autocorrelation is approximately:
test="AR1.mle"
, the MLE of the lag-1 autocorrelation is used, and its
standard deviation is estimated with the square root of the estimated variance
returned by arima
.
Bartels, R. (1982). The Rank Version of von Neumann's Ratio Test for Randomness. Journal of the American Statistical Association 77(377), 40--46.
Berthouex, P.M., and L.C. Brown. (2002). Statistics for Environmental Engineers. Second Edition. Lewis Publishers, Boca Raton, FL.
Box, G.E.P., and G.M. Jenkins. (1976). Time Series Analysis: Forecasting and Control. Prentice Hall, Englewood Cliffs, NJ, Chapter 2.
Cox, D.R. (1966). The Null Distribution of the First Serial Correlation Coefficient. Biometrika 53, 623--626.
Draper, N., and H. Smith. (1998). Applied Regression Analysis. Third Edition. John Wiley and Sons, New York, pp.69-70;181-192.
Durbin, J., and G.S. Watson. (1950). Testing for Serial Correlation in Least Squares Regression I. Biometrika 37, 409--428.
Durbin, J., and G.S. Watson. (1951). Testing for Serial Correlation in Least Squares Regression II. Biometrika 38, 159--178.
Durbin, J., and G.S. Watson. (1971). Testing for Serial Correlation in Least Squares Regression III. Biometrika 58, 1--19.
Helsel, D.R., and R.M. Hirsch. (1992). Statistical Methods in Water Resources Research. Elsevier, New York, NY, pp.250--253.
Johnson, N. L., S. Kotz, and N. Balakrishnan. (1995). Continuous Univariate Distributions, Volume 2. Second Edition. John Wiley and Sons, New York, Chapter 25.
Knoke, J.D. (1975). Testing for Randomness Against Autocorrelation Alternatives: The Parametric Case. Biometrika 62, 571--575.
Knoke, J.D. (1977). Testing for Randomness Against Autocorrelation Alternatives: Alternative Tests. Biometrika 64, 523--529.
Lehmann, E.L. (1975). Nonparametrics: Statistical Methods Based on Ranks. Holden-Day, Oakland, CA, 457pp.
von Neumann, J., R.H. Kent, H.R. Bellinson, and B.I. Hart. (1941). The Mean Square Successive Difference. Annals of Mathematical Statistics 12(2), 153--162.
Wald, A., and J. Wolfowitz. (1943). An Exact Test for Randomness in the Non-Parametric Case Based on Serial Correlation. Annals of Mathematical Statistics 14, 378--388.
htest.object
, acf
, ar
,
arima
, arima.sim
,
ts.plot
, plot.ts
,
lag.plot
, Hypothesis Tests.
# NOT RUN {
# Generate a purely random normal process, then use serialCorrelationTest
# to test for the presence of correlation.
# (Note: the call to set.seed allows you to reproduce this example.)
set.seed(345)
x <- rnorm(100)
# Look at the data
#-----------------
dev.new()
ts.plot(x)
dev.new()
acf(x)
# Test for serial correlation
#----------------------------
serialCorrelationTest(x)
#Results of Hypothesis Test
#--------------------------
#
#Null Hypothesis: rho = 0
#
#Alternative Hypothesis: True rho is not equal to 0
#
#Test Name: Rank von Neumann Test for
# Lag-1 Autocorrelation
# (Beta Approximation)
#
#Estimated Parameter(s): rho = 0.02773737
#
#Estimation Method: Yule-Walker
#
#Data: x
#
#Sample Size: 100
#
#Test Statistic: RVN = 1.929733
#
#P-value: 0.7253405
#
#Confidence Interval for: rho
#
#Confidence Interval Method: Normal Approximation
#
#Confidence Interval Type: two-sided
#
#Confidence Level: 95%
#
#Confidence Interval: LCL = -0.1681836
# UCL = 0.2236584
# Clean up
#---------
rm(x)
graphics.off()
#==========
# Now use the R function arima.sim to generate an AR(1) process with a
# lag-1 autocorrelation of 0.8, then test for autocorrelation.
set.seed(432)
y <- arima.sim(model = list(ar = 0.8), n = 100)
# Look at the data
#-----------------
dev.new()
ts.plot(y)
dev.new()
acf(y)
# Test for serial correlation
#----------------------------
serialCorrelationTest(y)
#Results of Hypothesis Test
#--------------------------
#
#Null Hypothesis: rho = 0
#
#Alternative Hypothesis: True rho is not equal to 0
#
#Test Name: Rank von Neumann Test for
# Lag-1 Autocorrelation
# (Beta Approximation)
#
#Estimated Parameter(s): rho = 0.835214
#
#Estimation Method: Yule-Walker
#
#Data: y
#
#Sample Size: 100
#
#Test Statistic: RVN = 0.3743174
#
#P-value: 0
#
#Confidence Interval for: rho
#
#Confidence Interval Method: Normal Approximation
#
#Confidence Interval Type: two-sided
#
#Confidence Level: 95%
#
#Confidence Interval: LCL = 0.7274307
# UCL = 0.9429973
#----------
# Clean up
#---------
rm(y)
graphics.off()
#==========
# The data frame Air.df contains information on ozone (ppb^1/3),
# radiation (langleys), temperature (degrees F), and wind speed (mph)
# for 153 consecutive days between May 1 and September 30, 1973.
# First test for serial correlation in (the cube root of) ozone.
# Note that we must use the test based on the MLE because the time series
# contains missing values. Serial correlation appears to be present.
# Next fit a linear model that includes the predictor variables temperature,
# radiation, and wind speed, and test for the presence of serial correlation
# in the residuals. There is no evidence of serial correlation.
# Look at the data
#-----------------
Air.df
# ozone radiation temperature wind
#05/01/1973 3.448217 190 67 7.4
#05/02/1973 3.301927 118 72 8.0
#05/03/1973 2.289428 149 74 12.6
#05/04/1973 2.620741 313 62 11.5
#05/05/1973 NA NA 56 14.3
#...
#09/27/1973 NA 145 77 13.2
#09/28/1973 2.410142 191 75 14.3
#09/29/1973 2.620741 131 76 8.0
#09/30/1973 2.714418 223 68 11.5
#----------
# Test for serial correlation
#----------------------------
with(Air.df,
serialCorrelationTest(ozone, test = "AR1.mle"))
#Results of Hypothesis Test
#--------------------------
#
#Null Hypothesis: rho = 0
#
#Alternative Hypothesis: True rho is not equal to 0
#
#Test Name: z-Test for
# Lag-1 Autocorrelation
# (Wald Test Based on MLE)
#
#Estimated Parameter(s): rho = 0.5641616
#
#Estimation Method: Maximum Likelihood
#
#Data: ozone
#
#Sample Size: 153
#
#Number NA/NaN/Inf's: 37
#
#Test Statistic: z = 7.586952
#
#P-value: 3.28626e-14
#
#Confidence Interval for: rho
#
#Confidence Interval Method: Normal Approximation
#
#Confidence Interval Type: two-sided
#
#Confidence Level: 95%
#
#Confidence Interval: LCL = 0.4184197
# UCL = 0.7099034
#----------
# Next fit a linear model that includes the predictor variables temperature,
# radiation, and wind speed, and test for the presence of serial correlation
# in the residuals. Note setting the argument na.action = na.exclude in the
# call to lm to correctly deal with missing values.
#----------------------------------------------------------------------------
lm.ozone <- lm(ozone ~ radiation + temperature + wind +
I(temperature^2) + I(wind^2),
data = Air.df, na.action = na.exclude)
# Now test for serial correlation in the residuals.
#--------------------------------------------------
serialCorrelationTest(lm.ozone, test = "AR1.mle")
#Results of Hypothesis Test
#--------------------------
#
#Null Hypothesis: rho = 0
#
#Alternative Hypothesis: True rho is not equal to 0
#
#Test Name: z-Test for
# Lag-1 Autocorrelation
# (Wald Test Based on MLE)
#
#Estimated Parameter(s): rho = 0.1298024
#
#Estimation Method: Maximum Likelihood
#
#Data: Residuals
#
#Data Source: lm.ozone
#
#Sample Size: 153
#
#Number NA/NaN/Inf's: 42
#
#Test Statistic: z = 1.285963
#
#P-value: 0.1984559
#
#Confidence Interval for: rho
#
#Confidence Interval Method: Normal Approximation
#
#Confidence Interval Type: two-sided
#
#Confidence Level: 95%
#
#Confidence Interval: LCL = -0.06803223
# UCL = 0.32763704
# Clean up
#---------
rm(lm.ozone)
# }
Run the code above in your browser using DataLab