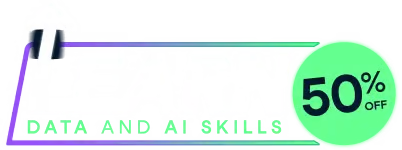
Last chance! 50% off unlimited learning
Sale ends in
updateModel.Res(Outcome,
covariates = "1",
pvalue = c(0.025, 0.05),
VarFrequencyTable,
variableList,
data,
type = c("LM", "LOGIT", "COX"),
testType=c("Binomial", "Wilcox", "tStudent"),
lastTopVariable = 0,
timeOutcome = "Time",
interaction = 1,
maxTrainModelSize = -1,
bootLoops=1)
data
that stores the variable to be predicted by the model
ranked.var
value returned by the ForwardSelection.Model.Res
function)
improvedResiduals
function: Binomial test ("Binomial"), Wilcoxon rank-sum test ("Wilcox"), Student's t-test ("tStudent"), or F-test ("Ftest")
data
that stores the time to event (needed only for a Cox proportional hazards regression model fitting)
lm
, glm
, or coxph
containing the final model
formula
with the formula used to fit the final model
testType
, for each feature found in the final model
updateModel.Bin
## Not run:
# # Start the graphics device driver to save all plots in a pdf format
# pdf(file = "Example.pdf")
# # Get the stage C prostate cancer data from the rpart package
# library(rpart)
# data(stagec)
# # Split the stages into several columns
# dataCancer <- cbind(stagec[,c(1:3,5:6)],
# gleason4 = 1*(stagec[,7] == 4),
# gleason5 = 1*(stagec[,7] == 5),
# gleason6 = 1*(stagec[,7] == 6),
# gleason7 = 1*(stagec[,7] == 7),
# gleason8 = 1*(stagec[,7] == 8),
# gleason910 = 1*(stagec[,7] >= 9),
# eet = 1*(stagec[,4] == 2),
# diploid = 1*(stagec[,8] == "diploid"),
# tetraploid = 1*(stagec[,8] == "tetraploid"),
# notAneuploid = 1-1*(stagec[,8] == "aneuploid"))
# # Remove the incomplete cases
# dataCancer <- dataCancer[complete.cases(dataCancer),]
# # Load a pre-stablished data frame with the names and descriptions of all variables
#
# data(cancerVarNames)
#
# # Rank the variables:
# # - Analyzing the raw data
# # - Using a Cox proportional hazards fitting
# # - According to the NeRI
# rankedDataCancer <- univariateRankVariables(variableList = cancerVarNames,
# formula = "Surv(pgtime, pgstat) ~ 1",
# Outcome = "pgstat",
# data = dataCancer,
# categorizationType = "Raw",
# type = "COX",
# rankingTest = "NeRI",
# description = "Description")
# # Get a Cox proportional hazards model using:
# # - 10 bootstrap loops
# # - The ranked variables
# # - The Wilcoxon rank-sum test as the feature inclusion criterion
# cancerModel <- ForwardSelection.Model.Res(loops = 10,
# Outcome = "pgstat",
# variableList = rankedDataCancer,
# data = dataCancer,
# type = "COX",
# testType= "Wilcox",
# timeOutcome = "pgtime")
# # Update the model, adding first order interactions
#
# uCancerModel <- updateModel.Res(Outcome = "pgstat",
# VarFrequencyTable = cancerModel$ranked.var,
# variableList = cancerVarNames,
# data = dataCancer,
# type = "COX",
# testType = "Wilcox",
# timeOutcome = "pgtime",
# interaction = 2)
# # Shut down the graphics device driver
# dev.off()## End(Not run)
Run the code above in your browser using DataLab