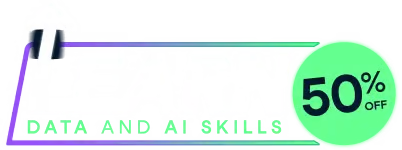
Last chance! 50% off unlimited learning
Sale ends in
Given a vector of initial estimates for the parameters, this function calculates the D-and PA- efficiency of a design
leff(
formula,
predvars,
parvars,
family = gaussian(),
inipars,
type = c("D", "PA"),
fimfunc = NULL,
x2,
w2,
x1,
w1,
npar = length(inipars),
prob = NULL
)
A vector of characters. Denotes the predictors in the formula
.
A vector of characters. Denotes the unknown parameters in the formula
.
A description of the response distribution and the link function to be used in the model.
This can be a family function, a call to a family function or a character string naming the family.
Every family function has a link argument allowing to specify the link function to be applied on the response variable.
If not specified, default links are used. For details see family
.
By default, a linear gaussian model gaussian()
is applied.
Vector. Initial values for the unknown parameters. It will be passed to the information matrix and also probability function.
A character. "D"
denotes the D-efficiency and "PA"
denotes the average P-efficiency.
A function. Returns the FIM as a matrix
. Required when formula
is missing. See 'Details' of minimax
.
Vector of design (support) points of the optimal design (x1
.
Vector of corresponding design weights for x2
.
Vector of design (support) points of leff
.
Vector of corresponding design weights for x1
.
Number of model parameters. Used when fimfunc
is given instead of formula
to specify the number of model parameters.
If not given, the sensitivity plot may be shifted below the y-axis. When NULL
, it will be set here to length(inipars)
.
Either formula
or a function
. When function, its argument are x
and param
, and they are the same as the arguments in fimfunc
.
prob
as a function takes the design points and vector of parameters and returns the probability of success at each design points.
See 'Examples'.
A value between 0 and 1.
For a known
The argument x1
is the vector of design points.
For design points with more than one dimension (the models with more than one predictors),
it is a concatenation of the design points, but dimension-wise.
For example, let the model has three predictors x1
is equal to
x = c(I1, I2, S1, S2, Z1, Z2)
.
McGree, J. M., Eccleston, J. A., and Duffull, S. B. (2008). Compound optimal design criteria for nonlinear models. Journal of Biopharmaceutical Statistics, 18(4), 646-661.
# NOT RUN {
p <- c(1, -2, 1, -1)
prior4.4 <- uniform(p -1.5, p + 1.5)
formula4.4 <- ~exp(b0+b1*x1+b2*x2+b3*x1*x2)/(1+exp(b0+b1*x1+b2*x2+b3*x1*x2))
prob4.4 <- ~1-1/(1+exp(b0 + b1 * x1 + b2 * x2 + b3 * x1 * x2))
predvars4.4 <- c("x1", "x2")
parvars4.4 <- c("b0", "b1", "b2", "b3")
# Locally D-optimal design is as follows:
## weight and point of D-optimal design
# Point1 Point2 Point3 Point4
# /1.00000 \ /-1.00000\ /0.06801 \ /1.00000 \
# \-1.00000/ \-1.00000/ \1.00000 / \1.00000 /
# Weight1 Weight2 Weight3 Weight4
# 0.250 0.250 0.250 0.250
xopt_D <- c(1, -1, .0680, 1, -1, -1, 1, 1)
wopt_D <- rep(.25, 4)
# Let see if we use only three of the design points, what is the relative efficiency.
leff(formula = formula4.4, predvars = predvars4.4, parvars = parvars4.4, family = binomial(),
x1 = c(1, -1, .0680, -1, -1, 1), w1 = c(.33, .33, .33),
inipars = p,
x2 = xopt_D, w2 = wopt_D)
# Wow, it heavily drops!
# Locally P-optimal design has only one support point and is -1 and 1
xopt_P <- c(-1, 1)
wopt_P <- 1
# What is the relative P-efficiency of the D-optimal design with respect to P-optimal design?
leff(formula = formula4.4, predvars = predvars4.4, parvars = parvars4.4, family = binomial(),
x1 = xopt_D, w1 = wopt_D,
inipars = p,
type = "PA",
prob = prob4.4,
x2 = xopt_P, w2 = wopt_P)
# .535
# }
Run the code above in your browser using DataLab