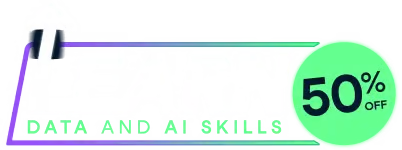
Last chance! 50% off unlimited learning
Sale ends in
sensitivity(actuals, predictedScores, threshold = 0.5)
data('ActualsAndScores')
sensitivity(actuals=ActualsAndScores$Actuals, predictedScores=ActualsAndScores$PredictedScores)
Run the code above in your browser using DataLab