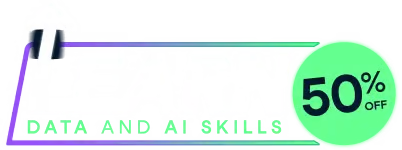
Last chance! 50% off unlimited learning
Sale ends in
mxFitFunctionR(fitfun, ..., units="-2lnL")
The 'fitfun' argument must be a function that accepts two arguments. The first argument is the mxModel that should be evaluated, and the second argument is some persistent state information that can be stored between one iteration of optimization to the next iteration. It is valid for the function to simply ignore the second argument.
The function must return either a single numeric value, or a list of exactly two elements. If the function returns a list, the first argument must be a single numeric value and the second element will be the new persistent state information to be passed into this function at the next iteration. The single numeric value will be used by the optimizer to perform optimization.
The initial default value for the persistant state information is NA.
Throwing an exception (via stop) from inside fitfun may result in unpredictable behavior. You may want to wrap your code in tryCatch while experimenting.
mxFitFunctionMultigroup
, mxFitFunctionML
,
mxFitFunctionWLS
, mxFitFunctionAlgebra
,
mxFitFunctionGREML
,
mxFitFunctionRow
More information about the OpenMx package may be found here.
# Create and fit a model using mxFitFunctionR
library(OpenMx)
A <- mxMatrix(nrow = 2, ncol = 2, values = c(1:4), free = TRUE, name = 'A')
squared <- function(x) { x ^ 2 }
# Define the objective function in R
objFunction <- function(model, state) {
values <- model$A$values
return(squared(values[1,1] - 4) + squared(values[1,2] - 3) +
squared(values[2,1] - 2) + squared(values[2,2] - 1))
}
# Define the expectation function
fitFunction <- mxFitFunctionR(objFunction)
# Define the model
tmpModel <- mxModel(model="exampleModel", A, fitFunction)
# Fit the model and print a summary
tmpModelOut <- mxRun(tmpModel)
summary(tmpModelOut)
Run the code above in your browser using DataLab