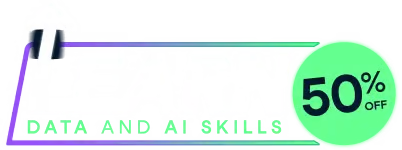
Last chance! 50% off unlimited learning
Sale ends in
RXpredict() makes in-sample predictions (i.e. computes "fitted.values") for all 6 forms of RXshrink estimation either at some user-specified m-Extent of Shrinkage, such as m=0.963, or at the Normal distribution-theory m-Extent most likely to achieve minimum Risk (minMSE).
RXpredict(x, data, m="minMSE", rscale=1)
An output list object of class RXpredict:
Predicted values for the "centered" and POSSIBLY "rescaled" outcome y-vector, cry. These values correspond, for example, to the default "predicted.values" from lm().
This the "centered" and POSSIBLY "rescaled" outcome y-vector from the input data.frame.
Predicted values for the Y-outcome variable, yvec.
The Y-outcome vector from the input data.frame specified by the "data" argument.
"numeric" Value of m-Extent implied by the call to RXpredict(), possibly via a default call with m="minMSE". Restriction: 0 <= m <= rank(X).
Observed m-Extent most close to the requested m-Extent AND is on the lattice of observed m-Extents stored within the given x-object.
An object output by one of the 6 RXshrink estimation functions. Thus class(x) must be "qm.ridge", "eff.ridge", "aug.lars", "uc.lars", "MLcalc" or "correct.signs".
Existing data.frame containing observations on all variables used by the RXshrink function for estimation of regression coefficients.
The m argument can be either [i] a single "numeric" value that is non-negative and does not exceed rank(X) or [ii] the (default) string "minMSE" to request use of the observed m-Extent of shrinkage most likely to be MSE optimal under Normal distribution-theory. For example, m="0.0" requests use of the (unbaised) OLS estimate [BLUE].
One of two possible choices (0 or 1) for "rescaling" of variables (after being "centered") to remove all "non-essential" ill-conditioning. Use "rscale=0" only when the RXshrink estimation function that computed the x-object also used "rscale=0". The default of "rscale=1" should be used in all other cases.
Bob Obenchain <wizbob@att.net>
Obenchain RL. (1978) Good and Optimal Ridge Estimators. Annals of Statistics 6, 1111-1121. tools:::Rd_expr_doi("10.1214/aos/1176344314")
Obenchain RL. (2005) Shrinkage Regression: ridge, BLUP, Bayes, spline and Stein. Electronic book-in-progress (185+ pages.) http://localcontrolstatistics.org
Obenchain RL. (2022) RXshrink_in_R.PDF RXshrink package vignette-like document, Version 2.2. http://localcontrolstatistics.org
qm.ridge
, eff.ridge
and MLtrue
.
data(tycobb)
form <- batavg~atbats+seasons+CMspl
rxefobj <- eff.ridge(form, data=tycobb)
tycfit <- RXpredict(rxefobj, tycobb, m="minMSE")
plot(tycfit)
tycobb$batavg[18] # Ty Cobb's batavg = 0.401 in 1922
abline(h=tycfit$cry[18], lty=2, lwd=3, col="red")
Run the code above in your browser using DataLab