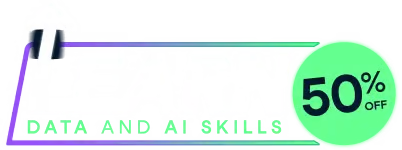
Last chance! 50% off unlimited learning
Sale ends in
Last chance! 50% off unlimited learning
Sale ends in
targetScore
should be used to obtain the probablistic score of miRNA target. The workhourse function is vbgmm
, which implementates multivariate variational Bayesian Gaussian mixture model.Bartel, D. P. (2009). MicroRNAs: Target Recognition and Regulatory Functions. Cell, 136(2), 215-233.
Bishop, C. M. (2006). Pattern recognition and machine learning. Springer, Information Science and Statistics. NY, USA. (p474-486)
targetScore
library(TargetScore)
ls("package:TargetScore")
Run the code above in your browser using DataLab