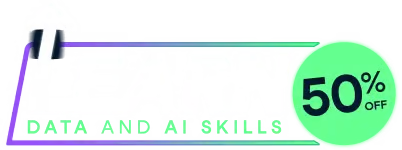
Last chance! 50% off unlimited learning
Sale ends in
multinomial(zero = NULL, parallel = FALSE, nointercept = NULL)
"vglmff"
(see vglmff-class
).
The object is used by modelling functions such as vglm
,
rrvglm
and vgam
.zero
and nointercept
can be inputted
with values that fail. For example, multinomial(zero=2,
nointercept=1:3)
means the second linear/additive predictor is
identically zero, which will cause a failure. Be careful about the use of other potentially contradictory constraints,
e.g., multinomial(zero=2, parallel = TRUE ~ x3)
. If in doubt,
apply constraints()
to the fitted object to check.
No check is made to verify that the response is nominal.
In almost all the literature, the constraint matrices associated
with this family of models are known. For example, setting
parallel=TRUE
will make all constraint matrices (except for
the intercept) equal to a vector of $M$ 1's. If the constraint
matrices are unknown and to be estimated, then this can be achieved
by fitting the model as a reduced-rank vector generalized linear model
(RR-VGLM; see rrvglm
). In particular, a multinomial logit
model with unknown constraint matrices is known as a stereotype model
(Anderson, 1984), and can be fitted with rrvglm
.
McCullagh, P. and Nelder, J. A. (1989) Generalized Linear Models, 2nd ed. London: Chapman & Hall.
Agresti, A. (2002) Categorical Data Analysis, 2nd ed. New York: Wiley.
Simonoff, J. S. (2003) Analyzing Categorical Data, New York: Springer-Verlag.
Anderson, J. A. (1984) Regression and ordered categorical variables. Journal of the Royal Statistical Society, Series B, Methodological, 46, 1--30.
Documentation accompanying the
acat
,
cumulative
,
cratio
,
sratio
,
dirichlet
,
dirmultinomial
,
rrvglm
,
Multinomial
,
iris
.# Example 1: fit a multinomial logit model to Edgar Anderson's iris data
data(iris)
fit = vglm(Species ~ ., multinomial, iris)
coef(fit, matrix=TRUE)
# Example 2a: a simple example
y = t(rmultinom(10, size = 20, prob=c(0.1,0.2,0.8))) # Counts
fit = vglm(y ~ 1, multinomial)
fitted(fit)[1:4,] # Proportions
fit@prior.weights # Not recommended for extraction of prior weights
weights(fit, type="prior", matrix=FALSE) # The better method
fit@y # Sample proportions
constraints(fit) # Constraint matrices
# Example 2b: Different input to Example 2a but same result
w = apply(y, 1, sum) # Prior weights
yprop = y / w # Sample proportions
fitprop = vglm(yprop ~ 1, multinomial, weights=w)
fitted(fitprop)[1:4,] # Proportions
weights(fitprop, type="prior", matrix=FALSE)
fitprop@y # Same as the input
# Example 3: Fit a rank-1 stereotype model
data(car.all)
fit = rrvglm(Country ~ Width + Height + HP, multinomial, car.all, Rank=1)
coef(fit) # Contains the C matrix
constraints(fit)$HP # The A matrix
coef(fit, matrix=TRUE) # The B matrix
Coef(fit)@C # The C matrix
ccoef(fit) # Better to get the C matrix this way
Coef(fit)@A # The A matrix
svd(coef(fit, matrix=TRUE)[-1,])$d # This has rank 1; = C %*% t(A)
# Example 4: The use of the xij argument (conditional logit model)
set.seed(111)
n = 100 # Number of people who travel to work
M = 3 # There are M+1 models of transport
ymat = matrix(0, n, M+1)
ymat[cbind(1:n, sample(x=M+1, size=n, replace=TRUE))] = 1
dimnames(ymat) = list(NULL, c("bus","train","car","walk"))
transport = data.frame(cost.bus=runif(n), cost.train=runif(n),
cost.car=runif(n), cost.walk=runif(n),
durn.bus=runif(n), durn.train=runif(n),
durn.car=runif(n), durn.walk=runif(n))
transport = round(transport, dig=2) # For convenience
transport = transform(transport,
Cost.bus = cost.bus - cost.walk,
Cost.car = cost.car - cost.walk,
Cost.train = cost.train - cost.walk,
Durn.bus = durn.bus - durn.walk,
Durn.car = durn.car - durn.walk,
Durn.train = durn.train - durn.walk)
fit = vglm(ymat ~ Cost.bus + Cost.train + Cost.car +
Durn.bus + Durn.train + Durn.car,
fam = multinomial,
xij = list(Cost ~ Cost.bus + Cost.train + Cost.car,
Durn ~ Durn.bus + Durn.train + Durn.car),
data=transport)
model.matrix(fit, type="lm")[1:7,] # LM model matrix
model.matrix(fit, type="vlm")[1:7,] # Big VLM model matrix
coef(fit)
coef(fit, matrix=TRUE)
coef(fit, matrix=TRUE, compress=FALSE)
summary(fit)
Run the code above in your browser using DataLab