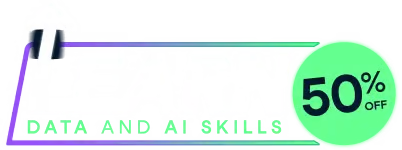
Last chance! 50% off unlimited learning
Sale ends in
vsmooth.spline(x, y, w = NULL, df = rep(5, M), spar = NULL, all.knots = FALSE,
iconstraint = diag(M), xconstraint = diag(M),
constraints = list("(Intercepts)" = diag(M), x = diag(M)),
var.arg = FALSE, scale.w = TRUE, nk = NULL,
control.spar = list())
x
component is used.
If a matrix, the first column is used.
x
may also be a complex vector, in which case
the real part is used, and the imaginary part is used for the response.y
component is used.
If a matrix, all but the first column is used.
In this help file, M
is the number of columns of y
if
there are no constraints on the functions.n
-row matrix
with the elements in matrix-band form (see iam
).
If a vector, then these are the number of observations.
By defaM
if there are no constraints), which
eqM
if there are no constraTRUE
then each distinct
value of x
will be a knot. By default, only a subset of
the unique values of x
are used; typically, the number
of knots is O(n^0.25)
for n
large,
but ifM
-row constraint matrix for the
intercepts. It must be of full column rank.
By default, the constraint matrix for the intercepts is the
M
by M
identity matrix, meaning no constraints.M
-row constraint matrix for x
.
It must be of full column rank.
By default, the constraint matrix for the intercepts is the
M
by M
identity matrix, meaning no constraints.iconstraint
and xconstraint
,
this is a list with two components corresponding to the
intercept and x
respectively. They must both be a
M
-row constraint matrix with full columw
are scaled so that the
diagonal elements have mean 1.all.knots
, and
must lie between 6 and n
+2 inclusive.smooth.spline
."vsmooth.spline"
(see
vsmooth.spline-class
).O(n M^3)
.
The argument spar
contains scaled smoothing parameters.
vsmooth.spline-class
,
plot.vsmooth.spline
,
predict.vsmooth.spline
,
iam
,
s
,
smooth.spline
.nn = 20; x = 2 + 5*(nn:1)/nn
x[2:4] = x[5:7] # Allow duplication
y1 = sin(x) + rnorm(nn, sd = 0.13)
y2 = cos(x) + rnorm(nn, sd = 0.13)
y3 = 1 + sin(x) + rnorm(nn, sd = 0.13) # Run this for constraints
y = cbind(y1, y2, y3)
ww = cbind(rep(3,nn), 4, (1:nn)/nn)
(fit = vsmooth.spline(x, y, w = ww, df = 5))
plot(fit) # The 1st and 3rd functions do not differ by a constant
mat = matrix(c(1,0,1, 0,1,0), 3, 2)
(fit2 = vsmooth.spline(x, y, w = ww, df = 5, iconstr = mat, xconstr = mat))
# The 1st and 3rd functions do differ by a constant:
mycols = c("orange", "blue", "orange")
plot(fit2, lcol = mycols, pcol = mycols, las = 1)
p = predict(fit, x = fit@x, deriv = 0)
max(abs(fit@y - with(p, y))) # Should be zero
par(mfrow = c(3, 1))
ux = seq(1, 8, len = 100)
for(d in 1:3) {
p = predict(fit, x = ux, deriv = d)
with(p, matplot(x, y, type = "l", main = paste("deriv =", d),
lwd = 2, ylab = "", cex.axis = 1.5,
cex.lab = 1.5, cex.main = 1.5))
}
Run the code above in your browser using DataLab