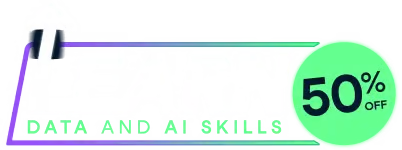
Last chance! 50% off unlimited learning
Sale ends in
dudi.acm
performs the multiple correspondence analysis of a factor table.
acm.burt
an utility giving the crossed Burt table of two factors table.
acm.disjonctif
an utility giving the complete disjunctive table of a factor table.
boxplot.acm
a graphic utility to interpret axes.
dudi.acm (df, row.w = rep(1, nrow(df)), scannf = TRUE, nf = 2)
acm.burt (df1, df2, counts = rep(1, nrow(df1)))
acm.disjonctif (df)
# S3 method for acm
boxplot(x, xax = 1, …)
data frames containing only factors
vector of row weights, by default, uniform weighting
a logical value indicating whether the eigenvalues bar plot should be displayed
if scannf FALSE, an integer indicating the number of kept axes
an object of class acm
the number of factor to display
further arguments passed to or from other methods
dudi.acm
returns a list of class acm
and dudi
(see dudi) containing
a data frame which rows are the variables, columns are the kept scores and the values are the correlation ratios
Tenenhaus, M. & Young, F.W. (1985) An analysis and synthesis of multiple correspondence analysis, optimal scaling, dual scaling, homogeneity analysis ans other methods for quantifying categorical multivariate data. Psychometrika, 50, 1, 91-119.
Lebart, L., A. Morineau, and M. Piron. 1995. Statistique exploratoire multidimensionnelle. Dunod, Paris.
# NOT RUN {
data(ours)
summary(ours)
if(adegraphicsLoaded()) {
g1 <- s1d.boxplot(dudi.acm(ours, scan = FALSE)$li[, 1], ours)
} else {
boxplot(dudi.acm(ours, scan = FALSE))
}
# }
# NOT RUN {
data(banque)
banque.acm <- dudi.acm(banque, scann = FALSE, nf = 3)
if(adegraphicsLoaded()) {
g2 <- adegraphics:::scatter.dudi(banque.acm)
} else {
scatter(banque.acm)
}
apply(banque.acm$cr, 2, mean)
banque.acm$eig[1:banque.acm$nf] # the same thing
if(adegraphicsLoaded()) {
g3 <- s1d.boxplot(banque.acm$li[, 1], banque)
g4 <- scatter(banque.acm)
} else {
boxplot(banque.acm)
scatter(banque.acm)
}
s.value(banque.acm$li, banque.acm$li[,3])
bb <- acm.burt(banque, banque)
bbcoa <- dudi.coa(bb, scann = FALSE)
plot(banque.acm$c1[,1], bbcoa$c1[,1])
# mca and coa of Burt table. Lebart & coll. section 1.4
bd <- acm.disjonctif(banque)
bdcoa <- dudi.coa(bd, scann = FALSE)
plot(banque.acm$li[,1], bdcoa$li[,1])
# mca and coa of disjonctive table. Lebart & coll. section 1.4
plot(banque.acm$co[,1], dudi.coa(bd, scann = FALSE)$co[,1])
# }
Run the code above in your browser using DataLab