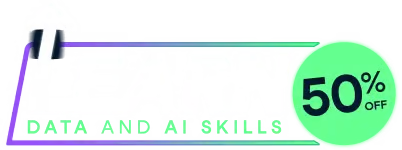
Last chance! 50% off unlimited learning
Sale ends in
Computes the impulse response coefficients of an object of class "bvar"
for
n.ahead
steps.
A plot function for objects of class "bvarirf".
irf(object, impulse = NULL, response = NULL, n.ahead = 5,
ci = 0.95, type = "feir", cumulative = FALSE)# S3 method for bvarirf
plot(x, ...)
an object of class "bvar"
, usually, a result of a call to
bvar
or bvec_to_bvar
.
name of the impulse variable.
name of the response variable.
number of steps ahead.
a numeric between 0 and 1 specifying the probability mass covered by the credible intervals. Defaults to 0.95.
type of the impulse resoponse. Possible choices are forecast error "feir"
(default), orthogonalised "oir"
, structural "sir"
, generalised "gir"
,
and structural generalised "sgir"
impulse responses.
logical specifying whether a cumulative IRF should be calculated.
an object of class "bvarirf", usually, a result of a call to irf
.
further graphical parameters.
A time-series object of class "bvarirf"
.
The function produces different types of impulse responses for the VAR model
Forecast error impulse responses
Orthogonalised impulse responses
Structural impulse responses
(Structural) Generalised impulse responses for variable "bvar"
object does not contain draws
of
L<U+00FC>tkepohl, H. (2007). New introduction to multiple time series analysis (2nd ed.). Berlin: Springer.
Pesaran, H. H., Shin, Y. (1998). Generalized impulse response analysis in linear multivariate models. Economics Letters, 58, 17-29.
# NOT RUN {
data("e1")
e1 <- diff(log(e1))
data <- gen_var(e1, p = 2, deterministic = "const")
y <- data$Y[, 1:73]
x <- data$Z[, 1:73]
set.seed(1234567)
iter <- 500 # Number of iterations of the Gibbs sampler
# Chosen number of iterations should be much higher, e.g. 30000.
burnin <- 100 # Number of burn-in draws
store <- iter - burnin
t <- ncol(y) # Number of observations
k <- nrow(y) # Number of endogenous variables
m <- k * nrow(x) # Number of estimated coefficients
# Set (uninformative) priors
a_mu_prior <- matrix(0, m) # Vector of prior parameter means
a_v_i_prior <- diag(0, m) # Inverse of the prior covariance matrix
u_sigma_df_prior <- 0 # Prior degrees of freedom
u_sigma_scale_prior <- diag(0, k) # Prior covariance matrix
u_sigma_df_post <- t + u_sigma_df_prior # Posterior degrees of freedom
# Initial values
u_sigma_i <- diag(.00001, k)
u_sigma <- solve(u_sigma_i)
# Data containers for posterior draws
draws_a <- matrix(NA, m, store)
draws_sigma <- matrix(NA, k^2, store)
# Start Gibbs sampler
for (draw in 1:iter) {
# Draw conditional mean parameters
a <- post_normal(y, x, u_sigma_i, a_mu_prior, a_v_i_prior)
# Draw variance-covariance matrix
u <- y - matrix(a, k) %*% x # Obtain residuals
u_sigma_scale_post <- solve(u_sigma_scale_prior + tcrossprod(u))
u_sigma_i <- matrix(rWishart(1, u_sigma_df_post, u_sigma_scale_post)[,, 1], k)
u_sigma <- solve(u_sigma_i) # Invert Sigma_i to obtain Sigma
# Store draws
if (draw > burnin) {
draws_a[, draw - burnin] <- a
draws_sigma[, draw - burnin] <- u_sigma
}
}
# Generate bvar object
bvar_est <- bvar(y = y, x = x, A = draws_a[1:18,],
C = draws_a[19:21, ], Sigma = draws_sigma)
# Generate impulse response
IR <- irf(bvar_est, impulse = "income", response = "cons", n.ahead = 8)
# Plot
plot(IR, main = "Forecast Error Impulse Response", xlab = "Period", ylab = "Response")
data("e1")
e1 <- diff(log(e1))
data <- gen_var(e1, p = 2, deterministic = "const")
y <- data$Y[, 1:73]
x <- data$Z[, 1:73]
set.seed(1234567)
iter <- 500 # Number of iterations of the Gibbs sampler
# Chosen number of iterations should be much higher, e.g. 30000.
burnin <- 100 # Number of burn-in draws
store <- iter - burnin
t <- ncol(y) # Number of observations
k <- nrow(y) # Number of endogenous variables
m <- k * nrow(x) # Number of estimated coefficients
# Set (uninformative) priors
a_mu_prior <- matrix(0, m) # Vector of prior parameter means
a_v_i_prior <- diag(0, m) # Inverse of the prior covariance matrix
u_sigma_df_prior <- 0 # Prior degrees of freedom
u_sigma_scale_prior <- diag(0, k) # Prior covariance matrix
u_sigma_df_post <- t + u_sigma_df_prior # Posterior degrees of freedom
# Initial values
u_sigma_i <- diag(.00001, k)
u_sigma <- solve(u_sigma_i)
# Data containers for posterior draws
draws_a <- matrix(NA, m, store)
draws_sigma <- matrix(NA, k^2, store)
# Start Gibbs sampler
for (draw in 1:iter) {
# Draw conditional mean parameters
a <- post_normal(y, x, u_sigma_i, a_mu_prior, a_v_i_prior)
# Draw variance-covariance matrix
u <- y - matrix(a, k) %*% x # Obtain residuals
u_sigma_scale_post <- solve(u_sigma_scale_prior + tcrossprod(u))
u_sigma_i <- matrix(rWishart(1, u_sigma_df_post, u_sigma_scale_post)[,, 1], k)
u_sigma <- solve(u_sigma_i) # Invert Sigma_i to obtain Sigma
# Store draws
if (draw > burnin) {
draws_a[, draw - burnin] <- a
draws_sigma[, draw - burnin] <- u_sigma
}
}
# Generate bvar object
bvar_est <- bvar(y = y, x = x, A = draws_a[1:18,],
C = draws_a[19:21, ], Sigma = draws_sigma)
# Generate impulse response
IR <- irf(bvar_est, impulse = "income", response = "cons", n.ahead = 8)
# Plot
plot(IR, main = "Forecast Error Impulse Response", xlab = "Period", ylab = "Response")
# }
Run the code above in your browser using DataLab