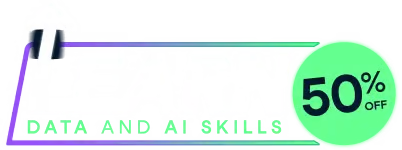
Last chance! 50% off unlimited learning
Sale ends in
bvhar
provides functions to analyze multivariate time series time
series using
Basically, the package focuses on the research with forecasting.
install.packages("bvhar")
You can install the development version from develop branch.
# install.packages("remotes")
remotes::install_github("ygeunkim/bvhar@develop")
We started to develop a Python version in python directory.
library(bvhar) # this package
library(dplyr)
Repeatedly, bvhar
is a research tool to analyze multivariate time
series model above
Model | function | prior |
---|---|---|
VAR | var_lm() | |
VHAR | vhar_lm() | |
BVAR | bvar_minnesota() | Minnesota (will move to var_bayes() ) |
BVHAR | bvhar_minnesota() | Minnesota (will move to vhar_bayes() ) |
BVAR | var_bayes() | SSVS, Horseshoe, Minnesota, NG, DL |
BVHAR | vhar_bayes() | SSVS, Horseshoe, Minnesota, NG, DL |
This readme document shows forecasting procedure briefly. Details about
each function are in vignettes and help documents. Note that each
bvar_minnesota()
and bvhar_minnesota()
will be integrated into
var_bayes()
and vhar_bayes()
and removed in the next version.
h-step ahead forecasting:
h <- 19
etf_split <- divide_ts(etf_vix, h) # Try ?divide_ts
etf_tr <- etf_split$train
etf_te <- etf_split$test
VAR(5):
mod_var <- var_lm(y = etf_tr, p = 5)
Forecasting:
forecast_var <- predict(mod_var, h)
MSE:
(msevar <- mse(forecast_var, etf_te))
#> GVZCLS OVXCLS VXFXICLS VXEEMCLS VXSLVCLS EVZCLS VXXLECLS VXGDXCLS
#> 5.381 14.689 2.838 9.451 10.078 0.654 22.436 9.992
#> VXEWZCLS
#> 10.647
mod_vhar <- vhar_lm(y = etf_tr)
MSE:
forecast_vhar <- predict(mod_vhar, h)
(msevhar <- mse(forecast_vhar, etf_te))
#> GVZCLS OVXCLS VXFXICLS VXEEMCLS VXSLVCLS EVZCLS VXXLECLS VXGDXCLS
#> 6.15 2.49 1.52 1.58 10.55 1.35 8.79 4.43
#> VXEWZCLS
#> 3.84
Minnesota prior:
lam <- .3
delta <- rep(1, ncol(etf_vix)) # litterman
sig <- apply(etf_tr, 2, sd)
eps <- 1e-04
(bvar_spec <- set_bvar(sig, lam, delta, eps))
#> Model Specification for BVAR
#>
#> Parameters: Coefficent matrice and Covariance matrix
#> Prior: Minnesota
#> ========================================================
#>
#> Setting for 'sigma':
#> GVZCLS OVXCLS VXFXICLS VXEEMCLS VXSLVCLS EVZCLS VXXLECLS VXGDXCLS
#> 3.77 10.63 3.81 4.39 5.99 2.27 4.88 7.45
#> VXEWZCLS
#> 7.03
#>
#> Setting for 'lambda':
#> [1] 0.3
#>
#> Setting for 'delta':
#> [1] 1 1 1 1 1 1 1 1 1
#>
#> Setting for 'eps':
#> [1] 1e-04
#>
#> Setting for 'hierarchical':
#> [1] FALSE
mod_bvar <- bvar_minnesota(y = etf_tr, p = 5, bayes_spec = bvar_spec)
MSE:
forecast_bvar <- predict(mod_bvar, h)
(msebvar <- mse(forecast_bvar, etf_te))
#> GVZCLS OVXCLS VXFXICLS VXEEMCLS VXSLVCLS EVZCLS VXXLECLS VXGDXCLS
#> 4.651 13.248 1.845 10.356 9.894 0.667 21.040 6.262
#> VXEWZCLS
#> 8.864
BVHAR-S:
(bvhar_spec_v1 <- set_bvhar(sig, lam, delta, eps))
#> Model Specification for BVHAR
#>
#> Parameters: Coefficent matrice and Covariance matrix
#> Prior: MN_VAR
#> ========================================================
#>
#> Setting for 'sigma':
#> GVZCLS OVXCLS VXFXICLS VXEEMCLS VXSLVCLS EVZCLS VXXLECLS VXGDXCLS
#> 3.77 10.63 3.81 4.39 5.99 2.27 4.88 7.45
#> VXEWZCLS
#> 7.03
#>
#> Setting for 'lambda':
#> [1] 0.3
#>
#> Setting for 'delta':
#> [1] 1 1 1 1 1 1 1 1 1
#>
#> Setting for 'eps':
#> [1] 1e-04
#>
#> Setting for 'hierarchical':
#> [1] FALSE
mod_bvhar_v1 <- bvhar_minnesota(y = etf_tr, bayes_spec = bvhar_spec_v1)
MSE:
forecast_bvhar_v1 <- predict(mod_bvhar_v1, h)
(msebvhar_v1 <- mse(forecast_bvhar_v1, etf_te))
#> GVZCLS OVXCLS VXFXICLS VXEEMCLS VXSLVCLS EVZCLS VXXLECLS VXGDXCLS
#> 3.199 6.067 1.471 5.142 5.946 0.878 12.165 2.553
#> VXEWZCLS
#> 6.462
BVHAR-L:
day <- rep(.1, ncol(etf_vix))
week <- rep(.1, ncol(etf_vix))
month <- rep(.1, ncol(etf_vix))
#----------------------------------
(bvhar_spec_v2 <- set_weight_bvhar(sig, lam, eps, day, week, month))
#> Model Specification for BVHAR
#>
#> Parameters: Coefficent matrice and Covariance matrix
#> Prior: MN_VHAR
#> ========================================================
#>
#> Setting for 'sigma':
#> GVZCLS OVXCLS VXFXICLS VXEEMCLS VXSLVCLS EVZCLS VXXLECLS VXGDXCLS
#> 3.77 10.63 3.81 4.39 5.99 2.27 4.88 7.45
#> VXEWZCLS
#> 7.03
#>
#> Setting for 'lambda':
#> [1] 0.3
#>
#> Setting for 'eps':
#> [1] 1e-04
#>
#> Setting for 'daily':
#> [1] 0.1 0.1 0.1 0.1 0.1 0.1 0.1 0.1 0.1
#>
#> Setting for 'weekly':
#> [1] 0.1 0.1 0.1 0.1 0.1 0.1 0.1 0.1 0.1
#>
#> Setting for 'monthly':
#> [1] 0.1 0.1 0.1 0.1 0.1 0.1 0.1 0.1 0.1
#>
#> Setting for 'hierarchical':
#> [1] FALSE
mod_bvhar_v2 <- bvhar_minnesota(y = etf_tr, bayes_spec = bvhar_spec_v2)
MSE:
forecast_bvhar_v2 <- predict(mod_bvhar_v2, h)
(msebvhar_v2 <- mse(forecast_bvhar_v2, etf_te))
#> GVZCLS OVXCLS VXFXICLS VXEEMCLS VXSLVCLS EVZCLS VXXLECLS VXGDXCLS
#> 3.63 3.85 1.64 5.12 5.75 1.08 13.60 2.58
#> VXEWZCLS
#> 5.54
Please cite this package with following BibTeX:
@Manual{,
title = {{bvhar}: Bayesian Vector Heterogeneous Autoregressive Modeling},
author = {Young Geun Kim and Changryong Baek},
year = {2023},
doi = {10.32614/CRAN.package.bvhar},
note = {R package version 2.1.0},
url = {https://cran.r-project.org/package=bvhar},
}
@Article{,
title = {Bayesian Vector Heterogeneous Autoregressive Modeling},
author = {Young Geun Kim and Changryong Baek},
journal = {Journal of Statistical Computation and Simulation},
year = {2024},
volume = {94},
number = {6},
pages = {1139--1157},
doi = {10.1080/00949655.2023.2281644},
}
Please note that the bvhar project is released with a Contributor Code of Conduct. By contributing to this project, you agree to abide by its terms.
install.packages('bvhar')