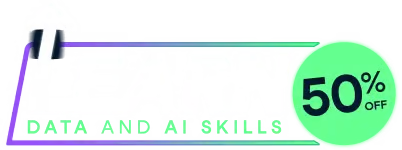
Last chance! 50% off unlimited learning
Sale ends in
Matrix of misclassification probabilities in a mixture distribution between two mixture components from estimated posterior probabilities regardless of component parameters, see Hennig (2010).
zmisclassification.matrix(z,pro=NULL,clustering=NULL,
ipairs="all",symmetric=TRUE,
stat="max")
matrix of posterior probabilities for observations (rows) to belong to mixture components (columns), so entries need to sum up to 1 for each row.
vector of component proportions, need to sum up to
1. Computed from z
as default.
vector of integers giving the estimated mixture
components for every observation. Computed from z
as
default.
"all"
or list of vectors of two integers. If
ipairs="all"
, computations are carried out for all pairs of
components. Otherwise, ipairs gives the pairs of components for
which computations are carried out.
logical. If TRUE
, the matrix is symmetrised,
see parameter stat
.
"max"
or "mean"
. The statistic by which the
two misclassification probabilities are aggregated if
symmetric=TRUE
.
A matrix with the (symmetrised, if required) misclassification
probabilities between each pair of mixture components. If
symmetric=FALSE
, matrix entry [i,j]
is the estimated
probability that an observation generated by component
j
is classified to component i
by maximum a posteriori rule.
Hennig, C. (2010) Methods for merging Gaussian mixture components, Advances in Data Analysis and Classification, 4, 3-34.
# NOT RUN {
set.seed(12345)
m <- rpois(20,lambda=5)
dim(m) <- c(5,4)
m <- m/apply(m,1,sum)
round(zmisclassification.matrix(m,symmetric=FALSE),digits=2)
# }
Run the code above in your browser using DataLab