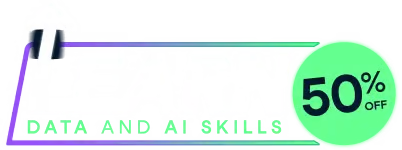
Last chance! 50% off unlimited learning
Sale ends in
An implementation of hyperparameter optimization for Gradient Boosted Trees on binary classification and regression problems. The current version provides two optimization methods: Bayesian optimization and random search.
# Load German credit data
data(german_credit)
train <- german_credit$train
test <- german_credit$test
target_idx <- german_credit$target_idx
pred_idx <- german_credit$pred_idx
# Train a GBT model with optimization on AUC
model <- gbts(train[, pred_idx], train[, target_idx], nitr = 200, pfmc = "auc")
# Predict on test data
prob_test <- predict(model, test[, pred_idx])
# Compute AUC on test data
comperf(test[, target_idx], prob_test, pfmc = "auc")
# Load Boston housing data
data(boston_housing)
train <- boston_housing$train
test <- boston_housing$test
target_idx <- boston_housing$target_idx
pred_idx <- boston_housing$pred_idx
# Train a GBT model with optimization on MSE
model <- gbts(train[, pred_idx], train[, target_idx], nitr = 200, pfmc = "mse")
# Predict on test data
prob_test <- predict(model, test[, pred_idx])
# Compute MSE on test data
comperf(test[, target_idx], prob_test, pfmc = "mse")
To get the current released version from CRAN:
install.packages("gbts")
To see a list of functions and datasets provided by gbts:
help(package = "gbts")
install.packages('gbts')