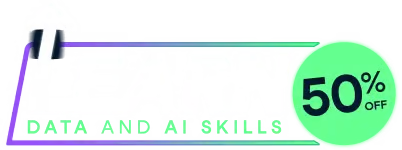
Last chance! 50% off unlimited learning
Sale ends in
Validate high-dimensional Cox models with time-dependent AUC
validate(
x,
time,
event,
model.type = c("lasso", "alasso", "flasso", "enet", "aenet", "mcp", "mnet", "scad",
"snet"),
alpha,
lambda,
pen.factor = NULL,
gamma,
lambda1,
lambda2,
method = c("bootstrap", "cv", "repeated.cv"),
boot.times = NULL,
nfolds = NULL,
rep.times = NULL,
tauc.type = c("CD", "SZ", "UNO"),
tauc.time,
seed = 1001,
trace = TRUE
)
Matrix of training data used for fitting the model; on which to run the validation.
Survival time.
Must be of the same length with the number of rows as x
.
Status indicator, normally 0 = alive, 1 = dead.
Must be of the same length with the number of rows as x
.
Model type to validate. Could be one of "lasso"
,
"alasso"
, "flasso"
, "enet"
, "aenet"
,
"mcp"
, "mnet"
, "scad"
, or "snet"
.
Value of the elastic-net mixing parameter alpha for
enet
, aenet
, mnet
, and snet
models.
For lasso
, alasso
, mcp
, and scad
models,
please set alpha = 1
.
alpha=1
: lasso (l1) penalty; alpha=0
: ridge (l2) penalty.
Note that for mnet
and snet
models,
alpha
can be set to very close to 0 but not 0 exactly.
Value of the penalty parameter lambda to use in the model fits on the resampled data. From the fitted Cox model.
Penalty factors to apply to each coefficient. From the fitted adaptive lasso or adaptive elastic-net model.
Value of the model parameter gamma for MCP/SCAD/Mnet/Snet models.
Value of the penalty parameter lambda1 for fused lasso model.
Value of the penalty parameter lambda2 for fused lasso model.
Validation method.
Could be "bootstrap"
, "cv"
, or "repeated.cv"
.
Number of repetitions for bootstrap.
Number of folds for cross-validation and repeated cross-validation.
Number of repeated times for repeated cross-validation.
Type of time-dependent AUC.
Including "CD"
proposed by Chambless and Diao (2006).,
"SZ"
proposed by Song and Zhou (2008).,
"UNO"
proposed by Uno et al. (2007).
Numeric vector. Time points at which to evaluate the time-dependent AUC.
A random seed for resampling.
Logical. Output the validation progress or not.
Default is TRUE
.
Chambless, L. E. and G. Diao (2006). Estimation of time-dependent area under the ROC curve for long-term risk prediction. Statistics in Medicine 25, 3474--3486.
Song, X. and X.-H. Zhou (2008). A semiparametric approach for the covariate specific ROC curve with survival outcome. Statistica Sinica 18, 947--965.
Uno, H., T. Cai, L. Tian, and L. J. Wei (2007). Evaluating prediction rules for t-year survivors with censored regression models. Journal of the American Statistical Association 102, 527--537.
data(smart)
x <- as.matrix(smart[, -c(1, 2)])[1:500, ]
time <- smart$TEVENT[1:500]
event <- smart$EVENT[1:500]
y <- survival::Surv(time, event)
fit <- fit_lasso(x, y, nfolds = 5, rule = "lambda.1se", seed = 11)
# Model validation by bootstrap with time-dependent AUC
# Normally boot.times should be set to 200 or more,
# we set it to 3 here only to save example running time.
val.boot <- validate(
x, time, event,
model.type = "lasso",
alpha = 1, lambda = fit$lambda,
method = "bootstrap", boot.times = 3,
tauc.type = "UNO", tauc.time = seq(0.25, 2, 0.25) * 365,
seed = 1010
)
# Model validation by 5-fold cross-validation with time-dependent AUC
val.cv <- validate(
x, time, event,
model.type = "lasso",
alpha = 1, lambda = fit$lambda,
method = "cv", nfolds = 5,
tauc.type = "UNO", tauc.time = seq(0.25, 2, 0.25) * 365,
seed = 1010
)
# Model validation by repeated cross-validation with time-dependent AUC
val.repcv <- validate(
x, time, event,
model.type = "lasso",
alpha = 1, lambda = fit$lambda,
method = "repeated.cv", nfolds = 5, rep.times = 3,
tauc.type = "UNO", tauc.time = seq(0.25, 2, 0.25) * 365,
seed = 1010
)
# bootstrap-based discrimination curves has a very narrow band
print(val.boot)
summary(val.boot)
plot(val.boot)
# k-fold cv provides a more strict evaluation than bootstrap
print(val.cv)
summary(val.cv)
plot(val.cv)
# repeated cv provides similar results as k-fold cv
# but more robust than k-fold cv
print(val.repcv)
summary(val.repcv)
plot(val.repcv)
# # Test fused lasso, SCAD, and Mnet models
#
# data(smart)
# x = as.matrix(smart[, -c(1, 2)])[1:500,]
# time = smart$TEVENT[1:500]
# event = smart$EVENT[1:500]
# y = survival::Surv(time, event)
#
# set.seed(1010)
# val.boot = validate(
# x, time, event, model.type = "flasso",
# lambda1 = 5, lambda2 = 2,
# method = "bootstrap", boot.times = 10,
# tauc.type = "UNO", tauc.time = seq(0.25, 2, 0.25) * 365,
# seed = 1010)
#
# val.cv = validate(
# x, time, event, model.type = "scad",
# gamma = 3.7, alpha = 1, lambda = 0.05,
# method = "cv", nfolds = 5,
# tauc.type = "UNO", tauc.time = seq(0.25, 2, 0.25) * 365,
# seed = 1010)
#
# val.repcv = validate(
# x, time, event, model.type = "mnet",
# gamma = 3, alpha = 0.3, lambda = 0.05,
# method = "repeated.cv", nfolds = 5, rep.times = 3,
# tauc.type = "UNO", tauc.time = seq(0.25, 2, 0.25) * 365,
# seed = 1010)
#
# print(val.boot)
# summary(val.boot)
# plot(val.boot)
#
# print(val.cv)
# summary(val.cv)
# plot(val.cv)
#
# print(val.repcv)
# summary(val.repcv)
# plot(val.repcv)
Run the code above in your browser using DataLab