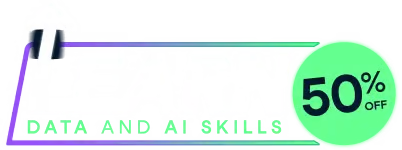
Last chance! 50% off unlimited learning
Sale ends in
prop
In the population, indicators of the same factor or latent variable have parallel correlations with all other variables. Of course, in the presence of sampling error, this parallelism will only be approximate. To assess this parallelism, proportionality coefficients are computed for each pair of variables in the input correlation matrix. Also output is a heat map of the resulting matrix of proportionality coefficients. Each graph is based on a default color theme. The original default is "blue", but other color palettes can be generated as well.
corProp(x=mycor,
main=NULL, heat.map=TRUE, bottom=3, right=3,
pdf.file=NULL, pdf.width=5, pdf.height=5)prop(...)
main=""
to turn off.TRUE
, display a heat map of the item
correlations with the diagonal ignored.In the current version the diagonal of the input correlation matrix is ignored. To maintain parallelism, the diagonal element of 1.00 would need to be replaced the corresponding communalities, which first requires a factor analysis.
Correlation
.# input correlation matrix of perfect two-factor model
# Factor Pattern for each Factor: 0.8, 0.6, 0.4
# Factor-Factor correlation: 0.3
mycor <- matrix(nrow=6, ncol=6, byrow=TRUE,
c(1.000,0.480,0.320,0.192,0.144,0.096,
0.480,1.000,0.240,0.144,0.108,0.072,
0.320,0.240,1.000,0.096,0.072,0.048,
0.192,0.144,0.096,1.000,0.480,0.320,
0.144,0.108,0.072,0.480,1.000,0.240,
0.096,0.072,0.048,0.320,0.240,1.000))
colnames(mycor) <- c("X1", "X2", "X3", "X4", "X5", "X6")
rownames(mycor) <- colnames(mycor)
# proportionality coefficients of correlation matrix mycor
# indicators of the same factor have proportional correlations
corProp()
# abbreviated form
prop()
# calculate and store proportionality coefficients in myprop
# order the proportionality coefficients to help identify factors
myprop <- corProp()
corReorder(myprop)
Run the code above in your browser using DataLab