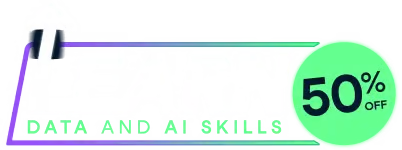
Last chance! 50% off unlimited learning
Sale ends in
Function qtree.moments
finds the expected value and variance for r
:th smallest observation in an iid sample of size n
from a population with a percentile-based distribution.
Function qtree.jointdens
computes the bivariate pdf for two quantiles
Function qtree.exy
approximates expected value of the product
Function qtree.varcov
returns the expected valuers, cumulative percentage values and the variance-covariance matrices
that correspond to given sample quantiles and underlying percentile-based distribution of the population.
Function interpolate.D
does a bilinear interpolation of the variance-covariance matrix of percentiles
that correspond to values F
of the cdf to values that correspond to values
ppi
.
qtree.moments(r,n,xi,F)
qtree.jointdens(x,r1,r2,n,xi,F)
qtree.exy(r1,r2,n,xi,F,npts=100)
qtree.varcov(obs,xi,F)
interpolate.D(D,ppi,F)
The ranks of the sample order statistics. r=1
means the smallest, r=n
the largest.
The sample size
The percentiles that specify the cdf in increasing order. The first element should be the population minimum and the last element should be the population maximum. A vector of same length as F
The values of the cdf that correspond to the percentiles of xi
. The first elements should be 0 and the last 1.
a matrix with two columns that gives the x-values for which the joint density is computed in qtree.jointdens
.
The number of regularly placed points that is used in the integral approximation of exy
.
A data frame of observed sample quantiles, possibly from several plots. The data frame should include
(at least) columns r
(the ranks), n
(sample size), plot
(plot id) and d
(observed diameter).
The rows should be ordered by r
within each plot, and all observations from same plot should follow each other.
The variance-covariance matrix of the residual errors (plot effects) of percentile models. The number of columns and rows should equal to the length of F
and xi
.
The values of cdf for which the covariances needs to be interpolated in interpolate.D
.
Function qtree.moments
returns a list with elements
The expected value of
The variance of
y gives the values of the pdf of plot(sol$x,sol$y,type="l")
.
Function qtree.exy returns a scalar, the approximate of E(X_{r1:n}X_{r2:n}).
Function qtree.varcov returns a list with elements
The original input data frame, augmented with the expected values in column Ed
and the corresponding values of the cdf of pEd
.
The variance-covariance matrix of the sample quantiles.
The original variance-covariance matrix, augmented with the variances and covariances
that correspond to the cdf values ppi
.
The values of cdf that correspond to the augmented matrix D
.
The variance-covariance matrix of the percentiles that correspond to the cdf values given in ppi
The covariance matrix between the percentiles that correspond to ppi
and F
Mehtatalo, L. 2005. Localizing a predicted diameter distribution using sample information. Forest Science 51(4): 292--302.
Mehtatalo, Lauri and Lappi, Juha 2020a. Biometry for Forestry and Environmental Data: with examples in R. New York: Chapman and Hall/CRC. 426 p. 10.1201/9780429173462
Mehtatalo, Lauri and Lappi, Juha 2020b. Biometry for Forestry and Environmental Data: with examples in R. Full Versions of The Web Examples. Available at http://www.biombook.org.
# NOT RUN {
F<-c(0,0.1,0.2,0.3,0.4,0.5,0.6,0.7,0.8,0.9,0.95,1)
# Predictions of logarithmic percentiles
xi<-c(1.638,2.352,2.646,2.792,2.91,2.996,3.079,3.151,3.234,3.349,3.417,3.593)
# The variance of their prediction errors
D<-matrix(c(0.161652909,0.050118692,0.022268974,0.010707222,0.006888751,0,
0.000209963,-0.002739361,-0.005478838,-0.00655718,-0.006718843,-0.009819052,
0.050118692,0.074627668,0.03492943,0.01564454,0.008771398,0,
-0.002691651,-0.005102312,-0.007290366,-0.008136685,-0.00817717,-0.009026883,
0.022268974,0.03492943,0.029281808,0.014958206,0.009351904,0,
-0.002646641,-0.003949305,-0.00592412,-0.006556639,-0.006993025,-0.007742731,
0.010707222,0.01564454,0.014958206,0.014182608,0.009328299,0,
-0.001525745,-0.002448765,-0.003571811,-0.004470387,-0.004791053,-0.005410252,
0.006888751,0.008771398,0.009351904,0.009328299,0.009799233,0,
-0.000925308,-0.001331631,-0.002491679,-0.003277911,-0.003514961,-0.003663479,
rep(0,12),
0.000209963,-0.002691651,-0.002646641,-0.001525745,-0.000925308,0,
0.003186033,0.003014887,0.002961818,0.003112953,0.003050486,0.002810937,
-0.002739361,-0.005102312,-0.003949305,-0.002448765,-0.001331631,0,
0.003014887,0.00592428,0.005843888,0.005793879,0.005971638,0.006247869,
-0.005478838,-0.007290366,-0.00592412,-0.003571811,-0.002491679,0,
0.002961818,0.005843888,0.00868157,0.008348973,0.008368812,0.008633202,
-0.00655718,-0.008136685,-0.006556639,-0.004470387,-0.003277911,0,
0.003112953,0.005793879,0.008348973,0.011040791,0.010962609,0.010906917,
-0.006718843,-0.00817717,-0.006993025,-0.004791053,-0.003514961,0,
0.003050486,0.005971638,0.008368812,0.010962609,0.013546621,0.013753718,
-0.009819052,-0.009026883,-0.007742731,-0.005410252,-0.003663479,0,
0.002810937,0.006247869,0.008633202,0.010906917,0.013753718,0.02496596),ncol=12)
# observed tree data, 5 trees from 2 plots
obs<-data.frame(r=c(1,3,6,1,2),n=c(7,7,7,9,9),plot=c(1,1,1,2,2),d=c(10,11,27,8,12))
# See Example 11.33 in Mehtatalo and Lappi 2020b
qtrees<-qtree.varcov(obs,xi,F)
obs<-qtrees$obs
mustar<-obs$Ed
ystar<-log(obs$d)
R<-qtrees$R
Dtayd<-interpolate.D(D,obs$pEd)
# }
Run the code above in your browser using DataLab