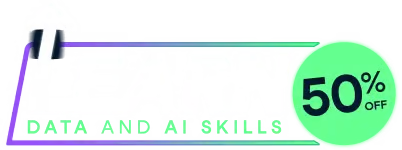
Last chance! 50% off unlimited learning
Sale ends in
Measure to compare true observed labels with predicted labels in multiclass classification tasks.
This Measure can be instantiated via the dictionary mlr_measures or with the associated sugar function msr()
:
mlr_measures$get("classif.mcc")
msr("classif.mcc")
Empty ParamSet
Type: "classif"
Range:
Minimize: FALSE
Required prediction: response
In the binary case, the Matthews Correlation Coefficient is defined as
In the multi-class case, the Matthews Correlation Coefficient is defined for a multi-class confusion matrix
The above formula is undefined if any of the four sums in the denominator is 0 in the binary case and more generally if either
When there are more than two classes, the MCC will no longer range between -1 and +1. Instead, the minimum value will be between -1 and 0 depending on the true distribution. The maximum value is always +1.
Dictionary of Measures: mlr_measures
as.data.table(mlr_measures)
for a complete table of all (also dynamically created) Measure implementations.
Other classification measures:
mlr_measures_classif.acc
,
mlr_measures_classif.auc
,
mlr_measures_classif.bacc
,
mlr_measures_classif.bbrier
,
mlr_measures_classif.ce
,
mlr_measures_classif.costs
,
mlr_measures_classif.dor
,
mlr_measures_classif.fbeta
,
mlr_measures_classif.fdr
,
mlr_measures_classif.fn
,
mlr_measures_classif.fnr
,
mlr_measures_classif.fomr
,
mlr_measures_classif.fp
,
mlr_measures_classif.fpr
,
mlr_measures_classif.logloss
,
mlr_measures_classif.mauc_au1p
,
mlr_measures_classif.mauc_au1u
,
mlr_measures_classif.mauc_aunp
,
mlr_measures_classif.mauc_aunu
,
mlr_measures_classif.mauc_mu
,
mlr_measures_classif.mbrier
,
mlr_measures_classif.npv
,
mlr_measures_classif.ppv
,
mlr_measures_classif.prauc
,
mlr_measures_classif.precision
,
mlr_measures_classif.recall
,
mlr_measures_classif.sensitivity
,
mlr_measures_classif.specificity
,
mlr_measures_classif.tn
,
mlr_measures_classif.tnr
,
mlr_measures_classif.tp
,
mlr_measures_classif.tpr
Other multiclass classification measures:
mlr_measures_classif.acc
,
mlr_measures_classif.bacc
,
mlr_measures_classif.ce
,
mlr_measures_classif.costs
,
mlr_measures_classif.logloss
,
mlr_measures_classif.mauc_au1p
,
mlr_measures_classif.mauc_au1u
,
mlr_measures_classif.mauc_aunp
,
mlr_measures_classif.mauc_aunu
,
mlr_measures_classif.mauc_mu
,
mlr_measures_classif.mbrier