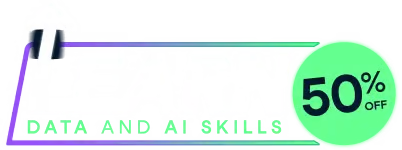
Last chance! 50% off unlimited learning
Sale ends in
The evalclust function calculates a set of evaluation criteria, see (Sulc et al., 2018) and provides the optimal number of clusters based on these criteria. It is primarily focused on the evaluation of hierarchical clustering results obtained by similarity measures different from the ones that occur in the nomclust package. Thus, it can serve for comparison of various similarity measures for categorical data.
evalclust(data, clusters)
A data.frame or a matrix with cases in rows and variables in colums.
A data.frame or a list of cluster memberships in a form of a sequence from the two-cluster solution to the maximal-cluster solution.
The function returns a list with two components.
The eval
component contains seven evaluation criteria in as vectors in a list. Namely, Within-cluster mutability coefficient (WCM), Within-cluster entropy coefficient (WCE),
Pseudo F Indices based on the mutability (PSFM) and the entropy (PSFE), Bayessian (BIC) and Akaike (AIC) information criteria for categorical data and the BK index.
To see them all in once, the form of a data.frame is more appropriate.
The opt
component is present in the output together with the eval
component. It displays the optimal number of clusters for the evaluation criteria from the eval
component, except for WCM and WCE, where the optimal number of clusters is based on the elbow method.
Sulc Z., Cibulkova J., Prochazka J., Rezankova H. (2018). Internal Evaluation Criteria for Categorical Data in Hierarchical Clustering: Optimal Number of Clusters Determination, Metodoloski Zveski, 15(2), p. 1-20.
# NOT RUN {
# sample data
data(data20)
# creating an object with results of hierarchical clustering
hca.object <- nomclust(data20, measure = "iof", method = "average", clu.high = 7)
# the cluster memberships
data20.clu <- hca.object$mem
# obtaining evaluation criteria for the provided dataset and cluster memberships
data20.eval <- evalclust(data20, clusters = data20.clu)
# }
Run the code above in your browser using DataLab