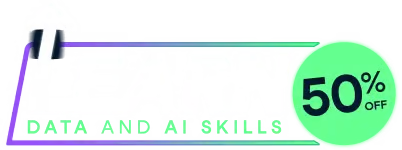
"predictSurvProb"(object,newdata,times,...)
"predictSurvProb"(object,newdata,times,...)
"predictSurvProb"(object,newdata,times,...)
"predictSurvProb"(object,newdata,times,...)
"predictSurvProb"(object,newdata,times,...)
"predictSurvProb"(object,newdata,times,...)
"predictSurvProb"(object,newdata,times,...)
"predictSurvProb"(object,newdata,times,...)
"predictSurvProb"(object,newdata,times,...)
"predictSurvProb"(object,newdata,times,...)
"predictSurvProb"(object,newdata,times,...)
"predictSurvProb"(object,newdata,times,...)
#' \method{predictSurvProb}{pecCtree}(object,newdata,times,...)
NROW(newdata)
and as many
columns as length(times)
. Each entry should be a probability and in
rows the values should be decreasing.
The function pec
requires survival probabilities for each row in
newdata at requested times. These probabilities are extracted from a fitted
model of class CLASS
with the function predictSurvProb.CLASS
.
Currently there are predictSurvProb
methods for objects of class cph
(library rms), coxph (library survival), aalen (library timereg), cox.aalen
(library timereg),
rpart (library rpart), product.limit (library prodlim),
survfit (library survival), psm (library rms)
predict
,survfit
# generate some survival data
library(prodlim)
set.seed(100)
d <- SimSurv(100)
# then fit a Cox model
library(rms)
coxmodel <- cph(Surv(time,status)~X1+X2,data=d,surv=TRUE)
# Extract predicted survival probabilities
# at selected time-points:
ttt <- quantile(d$time)
# for selected predictor values:
ndat <- data.frame(X1=c(0.25,0.25,-0.05,0.05),X2=c(0,1,0,1))
# as follows
predictSurvProb(coxmodel,newdata=ndat,times=ttt)
# stratified cox model
sfit <- coxph(Surv(time,status)~strata(X1)+X2,data=d,y=TRUE)
predictSurvProb(sfit,newdata=d[1:3,],times=c(1,3,5,10))
## simulate some learning and some validation data
learndat <- SimSurv(100)
valdat <- SimSurv(100)
## use the learning data to fit a Cox model
library(survival)
fitCox <- coxph(Surv(time,status)~X1+X2,data=learndat)
## suppose we want to predict the survival probabilities for all patients
## in the validation data at the following time points:
## 0, 12, 24, 36, 48, 60
psurv <- predictSurvProb(fitCox,newdata=valdat,times=seq(0,60,12))
## This is a matrix with survival probabilities
## one column for each of the 5 time points
## one row for each validation set individual
# Do the same for a randomSurvivalForest model
library(randomForestSRC)
rsfmodel <- rfsrc(Surv(time,status)~X1+X2,data=d)
predictSurvProb(rsfmodel,newdata=ndat,times=ttt)
## Cox with ridge option
f1 <- coxph(Surv(time,status)~X1+X2,data=learndat)
f2 <- coxph(Surv(time,status)~ridge(X1)+ridge(X2),data=learndat)
plot(predictSurvProb(f1,newdata=valdat,times=10),
pec:::predictSurvProb.coxph(f2,newdata=valdat,times=10),
xlim=c(0,1),
ylim=c(0,1),
xlab="Unpenalized predicted survival chance at 10",
ylab="Ridge predicted survival chance at 10")
Run the code above in your browser using DataLab