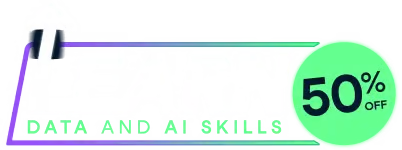
A package for learning optimal policies via doubly robust empirical welfare maximization over trees. This package implements the multi-action doubly robust approach of Zhou et al. (2018) in the case where we want to learn policies that belong to the class of depth k decision trees. Many practical policy applications require interpretable predictions. For example, a drug prescription guide that follows a simple 2-question Yes/No checklist can be encoded as a depth 2 decision tree (does the patient have a heart condition - etc.). policytree
currently has support for estimating multi-action treatment effects with one vs. all grf, calculating statistics such as double robust scores (support for a subset of grf forest types) and fitting optimal policies with exact tree search.
Some helpful links for getting started:
The R package documentation contains usage examples and method references.
For community questions and answers around usage, see the GitHub issues page.
Useful links:
# NOT RUN {
# Multi-action treatment effect estimation
n <- 250
p <- 10
X <- matrix(rnorm(n * p), n, p)
W <- sample(c("A", "B", "C"), n, replace = TRUE)
Y <- X[, 1] + X[, 2] * (W == "B") + X[, 3] * (W == "C") + runif(n)
multi.forest <- multi_causal_forest(X = X, Y = Y, W = W)
# tau.hats
predict(multi.forest)$predictions
# Policy learning
Gamma.matrix <- double_robust_scores(multi.forest)
train <- sample(1:n, 200)
opt.tree <- policy_tree(X[train, ], Gamma.matrix[train, ], depth = 2)
opt.tree
# Predict treatment on held out data
predict(opt.tree, X[-train, ])
# }
# NOT RUN {
# }
Run the code above in your browser using DataLab