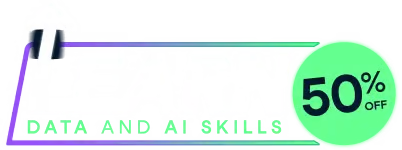
# S3 method for causal_forest
conditional_means(object, ...)# S3 method for instrumental_forest
conditional_means(object, ...)
# S3 method for multi_causal_forest
conditional_means(object, ...)
conditional_means(object, ...)
An appropriate causal forest type object
Additional arguments
A matrix of estimated mean rewards
causal_forest
: Mean rewards
instrumental_forest
: Mean rewards
multi_causal_forest
: Mean rewards
# NOT RUN {
# Compute conditional means for a multi_causal forest
n <- 500
p <- 10
X <- matrix(rnorm(n * p), n, p)
W <- sample(c("A", "B", "C"), n, replace = TRUE)
Y <- X[, 1] + X[, 2] * (W == "B") + X[, 3] * (W == "C") + runif(n)
forests <- multi_causal_forest(X = X, Y = Y, W = W)
mu.hats <- conditional_means(forests)
head(mu.hats)
# Compute conditional means for a causal forest
n <- 500
p <- 10
X <- matrix(rnorm(n * p), n, p)
W <- rbinom(n, 1, 0.5)
Y <- pmax(X[, 1], 0) * W + X[, 2] + pmin(X[, 3], 0) + rnorm(n)
c.forest <- grf::causal_forest(X, Y, W)
mu.hats <- conditional_means(c.forest)
# }
Run the code above in your browser using DataLab