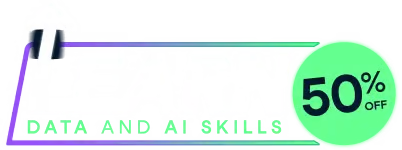
Last chance! 50% off unlimited learning
Sale ends in
VSS
) for estimating the optimal number of factors is plotted as a function of the increasing complexity and increasing number of factors.VSS.plot(x, plottitle = "Very Simple Structure", line = FALSE)
ICLUST
). Complexity 2 implies all except the greatest two, etc. Different complexities can suggest different number of optimal number of factors to extract. For personality items, complexity 1 and 2 are probably the most meaningful.
The Very Simple Structure criterion will tend to peak at the number of factors that are most interpretable for a given level of complexity. Note that some problems, the most interpretable number of factors will differ as a function of complexity. For instance, when doing the Harman 24 psychological variable problems, an unrotated solution of complexity one suggests one factor (g), while a complexity two solution suggests that a four factor solution is most appropriate. This latter probably reflects a bi-factor structure.
For examples of VSS.plot output, see
VSS
, ICLUST
, omega
#test.data <- Harman74.cor$cov
#my.vss <- VSS(test.data) #suggests that 4 factor complexity two solution is optimal
#VSS.plot(my.vss) #see the graphics window
## The function is currently defined as
function(x,plottitle="Very Simple Structure",line=FALSE)
{
n=dim(x)
symb=c(49,50,51,52) #plotting sym
plot(x$cfit.1,ylim=c(0,1),type="b",ylab="Very Simple Structure Fit",xlab="Number of Factors",pch=49)
if (line) lines(x$fit)
title(main=plottitle)
x$cfit.2[1]<-NA
x$cfit.3[1]<-NA
x$cfit.3[2]<-NA
x$cfit.4[1]<-NA
x$cfit.4[2]<-NA
x$cfit.4[3]<-NA
lines(x$cfit.2)
points(x$cfit.2,pch=50)
lines(x$cfit.3)
points(x$cfit.3,pch=symb[3])
lines(x$cfit.4)
points(x$cfit.4,pch=symb[4])
}
Run the code above in your browser using DataLab