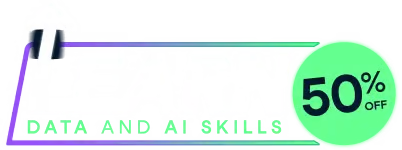
Last chance! 50% off unlimited learning
Sale ends in
x
is a RasterLayer, fun
is typically a function that can take a single vector as input, and return a vector of values of the same length (e.g. sqrt
). If x
is a RasterStack or RasterBrick, fun should operate on a vector of values (one vector for each cell). calc
returns a RasterLayer if fun
returns a single value (e.g. sum
) and it returns a RasterBrick if fun
returns more than one number, e.g., fun=quantile
.
In many cases, what can be achieved with calc
, can also be accomplished with a more intuitive 'raster-algebra' notation (see Arith-methods
). For example, r <- r * 2
instead of
r <- calc(r, fun=function(x){x * 2}
, or r <- sum(s)
instead of
r <- calc(s, fun=sum)
. However, calc
should be faster when using complex formulas on large datasets. With calc
it is possible to set an output filename and file type preferences.
See (overlay
) to use functions that refer to specific layers, like (function(a,b,c){a + sqrt(b) / c}
)## S3 method for class 'Raster,function':
calc(x, fun, filename='', na.rm, forcefun=FALSE, forceapply=FALSE, ...)
NA
values, if supported by 'fun' (only relevant when summarizing a multilayer Raster object into a RasterLayer)calc
to not use fun with applycalc
to use fun with applywriteRaster
overlay
, reclassify
, Arith-methods, Math-methodsr <- raster(ncols=36, nrows=18)
r[] <- 1:ncell(r)
# multiply values with 10
fun <- function(x) { x * 10 }
rc1 <- calc(r, fun)
# set values below 100 to NA.
fun <- function(x) { x[x<100] <- NA; return(x) }
rc2 <- calc(r, fun)
# set NA values to -9999
fun <- function(x) { x[is.na(x)] <- -9999; return(x)}
rc3 <- calc(rc2, fun)
# using a RasterStack as input
s <- stack(r, r*2, sqrt(r))
# return a RasterLayer
rs1 <- calc(s, sum)
# return a RasterBrick
rs2 <- calc(s, fun=function(x){x * 10})
# recycling by layer
rs3 <- calc(s, fun=function(x){x * c(1, 5, 10)})
# use overlay when you want to refer to indiviudal layer in the function
# but it can be done with calc:
rs4 <- calc(s, fun=function(x){x[1]+x[2]*x[3]})
##
# Some regression examples
##
# create data
r <- raster(nrow=10, ncol=10)
s1 <- s2<- list()
for (i in 1:12) {
s1[i] <- setValues(r, rnorm(ncell(r), i, 3) )
s2[i] <- setValues(r, rnorm(ncell(r), i, 3) )
}
s1 <- stack(s1)
s2 <- stack(s2)
# regression of values in one brick (or stack) with another
s <- stack(s1, s2)
# s1 and s2 have 12 layers; coefficients[2] is the slope
fun <- function(x) { lm(x[1:12] ~ x[13:24])$coefficients[2] }
x1 <- calc(s, fun)
# regression of values in one brick (or stack) with 'time'
time <- 1:nlayers(s)
fun <- function(x) { lm(x ~ time)$coefficients[2] }
x2 <- calc(s, fun)
# get multiple layers, e.g. the slope _and_ intercept
fun <- function(x) { lm(x ~ time)$coefficients }
x3 <- calc(s, fun)
Run the code above in your browser using DataLab