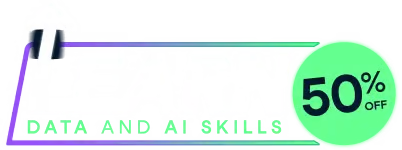
step_select()
creates a specification of a recipe step that will select
variables using dplyr::select()
.
step_select(
recipe,
...,
role = NA,
trained = FALSE,
skip = FALSE,
id = rand_id("select")
)
An updated version of recipe
with the new step added to the
sequence of any existing operations.
A recipe object. The step will be added to the sequence of operations for this recipe.
One or more selector functions to choose variables
for this step. See selections()
for more details.
For model terms selected by this step, what analysis role should they be assigned?
A logical to indicate if the quantities for preprocessing have been estimated.
A logical. Should the step be skipped when the
recipe is baked by bake()
? While all operations are baked
when prep()
is run, some operations may not be able to be
conducted on new data (e.g. processing the outcome variable(s)).
Care should be taken when using skip = TRUE
as it may affect
the computations for subsequent operations.
A character string that is unique to this step to identify it.
When you tidy()
this step, a tibble with column
terms
which contains the select
expressions as character strings
(and are not reparsable) is returned.
The underlying operation does not allow for case weights.
When an object in the user's global environment is
referenced in the expression defining the new variable(s),
it is a good idea to use quasiquotation (e.g. !!
) to embed
the value of the object in the expression (to be portable
between sessions). See the examples.
This step can potentially remove columns from the data set. This may cause issues for subsequent steps in your recipe if the missing columns are specifically referenced by name. To avoid this, see the advice in the Tips for saving recipes and filtering columns section of selections.
Other variable filter steps:
step_corr()
,
step_filter_missing()
,
step_lincomb()
,
step_nzv()
,
step_rm()
,
step_zv()
Other dplyr steps:
step_arrange()
,
step_filter()
,
step_mutate_at()
,
step_mutate()
,
step_rename_at()
,
step_rename()
,
step_sample()
,
step_slice()
library(dplyr)
iris_tbl <- as_tibble(iris)
iris_train <- slice(iris_tbl, 1:75)
iris_test <- slice(iris_tbl, 76:150)
dplyr_train <- select(iris_train, Species, starts_with("Sepal"))
dplyr_test <- select(iris_test, Species, starts_with("Sepal"))
rec <- recipe(~., data = iris_train) %>%
step_select(Species, starts_with("Sepal")) %>%
prep(training = iris_train)
rec_train <- bake(rec, new_data = NULL)
all.equal(dplyr_train, rec_train)
rec_test <- bake(rec, iris_test)
all.equal(dplyr_test, rec_test)
# Local variables
sepal_vars <- c("Sepal.Width", "Sepal.Length")
qq_rec <-
recipe(~., data = iris_train) %>%
# fine for interactive usage
step_select(Species, all_of(sepal_vars)) %>%
# best approach for saving a recipe to disk
step_select(Species, all_of(!!sepal_vars))
# Note that `sepal_vars` is inlined in the second approach
qq_rec
Run the code above in your browser using DataLab