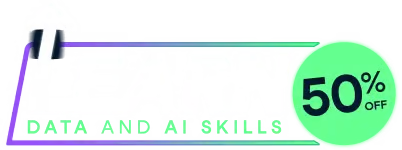
adore.filter(y, p.test = 15, minNonNAs = 5,
min.width = 11, max.width = 100, width.search="geometric",
rtr=2, extrapolate=TRUE, calc.qn = FALSE)
adore.filter
returns an object of class adore.filter
.
An object of class adore.filter
is a list containing the
following components:t
, the window width was not reduced, the entry level.list[[t]]
simply corresponds to level[t]
.
However, if more than one iteration took place, level.list[[t]]
is a vector which contains all level estimations which were
evaluated until the final estimate mu[t]
passed the goodness of fit test and was stored.level.list
.level.list
and slope.list
.calc.qn = TRUE
, else sigma
does not exist).y
, and the settings used for the analysis are
returned as the list members min.width
, max.width
, width.search
,
p.test
, minNonNAs
, rtr
, extrapolate
, and calc.qn
.
Application of the function plot
to an object of class
aoRM
returns a plot showing the original time series
with the filtered output.adore.filter
works by applying Repeated Median (RM)
regression (Siegel, 1982) to a moving time window with a length varying between
min.width
and max.width
.
For each point in time, the window width is adapted to the current data situation by a goodness of fit test for
the most recent signal level estimation.
The test uses the absolute value of the sum of the RM residuals in the subset specified by p.test
.
The critical value for the test decision corresponds to a slightly modified 0.95-quantile of the
distribution of the test statistic and is stored in the data set critvals
.
A more detailed description of the filter can be found in
Schettlinger, Fried, Gather (2008).robreg.filter
, wrm.filter
, madore.filter
.# # # # # # # # # #
# Short and noise-free time series
series <- c(rep(0,30),rep(10,30),seq(10,5,length=20),seq(5,15,length=20))
# Adaptive online signal extraction without & with 'restrict to range' rule
t.without.rtr <- adore.filter(series, rtr=0)
plot(t.without.rtr)
t.with.rtr1 <- adore.filter(series, rtr=1)
lines(t.with.rtr1$level, col="blue")
t.with.rtr2 <- adore.filter(series)
lines(t.with.rtr2$level, col="green3",lty=2)
legend("top",c("Signal with rtr=1","Signal with rtr=2"),col=c("blue","green3"),lty=c(1,2),bty="n")
# # # # # # # # # #
# Short and noise-free time series + 1 outlier
ol.series <- series
ol.series[63] <- 3
# Adaptive online signal extraction without & with 'restrict to range' rule
t.without.rtr <- adore.filter(ol.series, rtr=0)
plot(t.without.rtr)
t.with.rtr1 <- adore.filter(ol.series, rtr=1)
lines(t.with.rtr1$level, col="blue")
t.with.rtr2 <- adore.filter(ol.series)
lines(t.with.rtr2$level, col="green3",lty=2)
legend("top",c("Signal with rtr=1","Signal with rtr=2"),col=c("blue","green3"),lty=c(1,2),bty="n")
# # # # # # # # # #
# Noisy time series with level shifts, trend changes and shifts in the scale of the error term
true.signal <- c(rep(0,150),rep(10,150),seq(10,5,length=100),seq(5,15,length=100))
series2 <- true.signal + c(rnorm(250,sd=1), rnorm(200,sd=3), rnorm(50,sd=1))
# Adaptive online signal extraction with additional Qn scale estimation
s2 <- adore.filter(series2, calc.qn=TRUE)
par(mfrow=c(3,1))
plot(s2)
plot(s2$sigma,type="l",main="Corresponding Qn Scale Estimation",ylab="sigma",xlab="time")
lines(c(rep(1,250),rep(3,200),rep(1,150)),col="grey")
legend("topleft",c("True scale","Qn"),lty=c(1,1),col=c("grey","black"),bty="n")
plot(s2$width,type="l",main="Corresponding Window Width",ylab="width",xlab="time")
Run the code above in your browser using DataLab