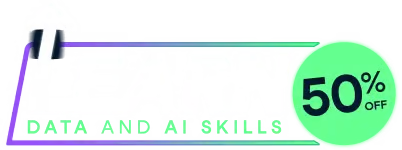
Last chance! 50% off unlimited learning
Sale ends in
pcc
computes the Partial Correlation Coefficients (PCC), or
Partial Rank Correlation Coefficients (PRCC), which are sensitivity
indices based on linear (resp. monotonic) assumptions, in the case of
(linearly) correlated factors.pcc(X, y, rank = FALSE, nboot = 0, conf = 0.95)
## S3 method for class 'pcc':
print(x, \dots)
## S3 method for class 'pcc':
plot(x, ylim = c(-1,1), ...)
as.data.frame
)
containing the design of experiments (model input variables).TRUE
, the analysis is done on the
ranks.pcc
.par
).pcc
returns a list of class "pcc"
, containing the following
components:rank = TRUE
).rank = TRUE
).src
# a 100-sample with X1 ~ U(0.5, 1.5)
# X2 ~ U(1.5, 4.5)
# X3 ~ U(4.5, 13.5)
library(boot)
n <- 100
X <- data.frame(X1 = runif(n, 0.5, 1.5),
X2 = runif(n, 1.5, 4.5),
X3 = runif(n, 4.5, 13.5))
# linear model : Y = X1 + X2 + X3
y <- with(X, X1 + X2 + X3)
# sensitivity analysis
x <- pcc(X, y, nboot = 100)
print(x)
#plot(x) # TODO: find another example...
Run the code above in your browser using DataLab