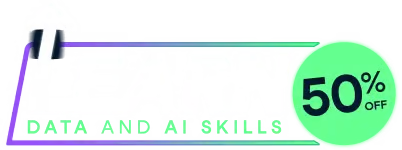
Last chance! 50% off unlimited learning
Sale ends in
This function animates the process of generating variates via acceptance-rejection for a specified density function (pdf) bounded by a specified majorizing function.
accrej(
n = 20,
pdf = function(x) dbeta(x, 3, 2),
majorizingFcn = NULL,
majorizingFcnType = NULL,
support = c(0, 1),
seed = NA,
maxTrials = Inf,
plot = TRUE,
showTitle = TRUE,
plotDelay = plot * -1
)
number of variates to generate.
desired probability density function from which random variates are to be drawn
majorizing function. Default value is NULL, corresponding to a constant majorizing function that is 1.01 times the maximum value of the pdf. May alternatively be provided as a user-specified function, or as a data frame requiring additional notation as either piecewise-constant or piecewise-linear. See examples.
used to indicate whether a majorizing function that
is provided via data frame is to be interpreted as
either piecewise-constant ("pwc"
) or
piecewise-linear ("pwl"
). If the majorizing
function is either the default or a user-specified
function (closure), the value of this parameter is
ignored.
the lower and upper bounds of the support of the probability distribution of interest, specified as a two-element vector.
initial seed for the uniform variates used during generation.
maximum number of accept-reject trials; infinite by default
if TRUE, visual display will be produced. If FALSE, generated variates will be returned without visual display.
if TRUE, display title in the main plot.
wait time, in seconds, between plots; -1 (default) for interactive mode, where the user is queried for input to progress.
Returns the n generated variates accepted.
There are three modes for visualizing the acceptance-rejection algorithm for generating random variates from a particular probability distribution:
interactive advance (plotDelay = -1
), where
pressing the 'ENTER' key advances to the next step
(an accepted random variate) in the algorithm,
typing 'j #' jumps ahead # steps,
typing 'q' quits immediately,
and typing 'e' proceeds to the end;
automatic advance (plotDelay
> 0); or
final visualization only (plotDelay = 0
).
As an alternative to visualizing, variates can be generated
# NOT RUN {
accrej(n = 20, seed = 8675309, plotDelay = 0)
# }
# NOT RUN {
accrej(n = 10, seed = 8675309, plotDelay = 0.1)
accrej(n = 10, seed = 8675309, plotDelay = -1)
# Piecewise-constant majorizing function
m <- function(x) {
if (x < 0.3) 1.0
else if (x < 0.85) 2.5
else 1.5
}
accrej(n = 100, seed = 8675309, majorizingFcn = m, plotDelay = 0)
# Piecewise-constant majorizing function as data frame
m <- data.frame(
x = c(0.0, 0.3, 0.85, 1.0),
y = c(1.0, 1.0, 2.5, 1.5))
accrej(n = 100, seed = 8675309, majorizingFcn = m,
majorizingFcnType = "pwc", plotDelay = 0)
# Piecewise-linear majorizing function as data frame
m <- data.frame(
x = c(0.0, 0.1, 0.3, 0.5, 0.7, 1.0),
y = c(0.0, 0.5, 1.1, 2.2, 1.9, 1.0))
accrej(n = 100, seed = 8675309, majorizingFcn = m,
majorizingFcnType = "pwl", plotDelay = 0)
# invalid majorizing function; should give warning
accrej(n = 20, majorizingFcn = function(x) dbeta(x, 1, 3), plotDelay = 0)
# }
# NOT RUN {
# Piecewise-linear majorizing function with power-distribution density function
m <- data.frame(x = c(0, 1, 2), y = c(0, 0.375, 1.5))
samples <- accrej(n = 100, pdf = function(x) (3 / 8) * x ^ 2, support = c(0,2),
majorizingFcn = m, majorizingFcnType = "pwl", plotDelay = 0)
# }
Run the code above in your browser using DataLab