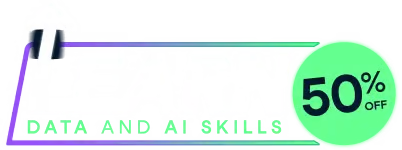
Last chance! 50% off unlimited learning
Sale ends in
NA
).set_na(x, values, as.attr = FALSE)
data.frame
or list
of variables where new
missing values should be defined. If x
is a data.frame
, each
column is assumed to be a new variable, where missings should be defined.NA
's.
Thus, for each variable in x
, values
are replaced by NA
's.
Or: a logical vector describing which values shouldTRUE
, values
of x
will not
be converted to NA
. Rather, the missing code values of values
will be added as missing-attribute is_na
x
, where each value of values
is replaced by an NA
.set_na
converts those values to NA
that are
specified in the function's values
argument; hence,
by default, set_na
ignores any missing code attributes
like is_na
. to_na
, by contrast, converts
values to NA
, which are defined as missing through the
is_na
-attribute of a vector (see labelled
).
If as.attr = TRUE
, values
in x
will not
be converted to NA
. Instead, the attribute is_na
will be added to x
, indicating which values should be coded
as missing. values
may either be numeric, with each number
indicating a value that should be defined as missing; or a vector
of logicals, describing which values should be translated to
missing values (see 'Examples').
Furthermore, see 'Details' in get_na
.replace_na
to replace NA
's with specific
values, rec
for general recoding of variables and
recode_to
for re-shifting value ranges. See
get_na
to get values of missing values in
labelled vectors and to_na
to convert missing value
codes into NA
.# create random variable
dummy <- sample(1:8, 100, replace = TRUE)
# show value distribution
table(dummy)
# set value 1 and 8 as missings
dummy <- set_na(dummy, c(1, 8))
# show value distribution, including missings
table(dummy, exclude = NULL)
# create sample data frame
dummy <- data.frame(var1 = sample(1:8, 100, replace = TRUE),
var2 = sample(1:10, 100, replace = TRUE),
var3 = sample(1:6, 100, replace = TRUE))
# show head of data frame
head(dummy)
# set value 2 and 4 as missings
dummy <- set_na(dummy, c(2, 4))
# show head of new data frame
head(dummy)
# create list of variables
data(efc)
dummy <- list(efc$c82cop1, efc$c83cop2, efc$c84cop3)
# check original distribution of categories
lapply(dummy, table, exclude = NULL)
# set 3 to NA
lapply(set_na(dummy, 3), table, exclude = NULL)
# create random variable
dummy <- sample(1:5, 100, replace = TRUE)
# declare missing values, but only as attribute
dummy <- set_na(dummy, c(3, 5), as.attr = TRUE)
str(dummy)
table(dummy)
get_na(dummy)
# create random variable
dummy <- sample(1:5, 100, replace = TRUE)
# declare missing values, but only as attribute
# missing code definition may be logical indices
dummy <- set_na(dummy,
c(FALSE, FALSE, FALSE, TRUE, TRUE),
as.attr = TRUE)
str(dummy)
table(dummy)
get_na(dummy)
Run the code above in your browser using DataLab