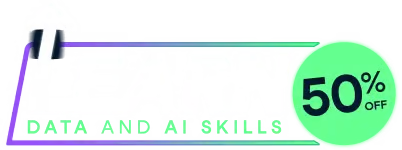
Last chance! 50% off unlimited learning
Sale ends in
Most functions to fit multilevel and mixed effects models only
allow to specify frequency weights, but not design (i.e. sampling or probability)
weights, which should be used when analyzing complex samples and survey data.
scale_weights()
implements an algorithm proposed by Aaparouhov (2006)
and Carle (2009) to rescale design weights in survey data to account for
the grouping structure of multilevel models, which then can be used for
multilevel modelling.
scale_weights(x, cluster.id, pweight)
A data frame.
Variable indicating the grouping structure (strata) of the survey data (level-2-cluster variable).
Variable indicating the probability (design or sampling) weights of the survey data (level-1-weight).
x
, with two new variables: svywght_a
and svywght_b
,
which represent the rescaled design weights to use in multilevel models.
Rescaling is based on two methods: For svywght_a
, the sample
weights pweight
are adjusted by a factor that represents the proportion
of cluster size divided by the sum of sampling weights within each cluster.
The adjustment factor for svywght_b
is the sum of sample weights
within each cluster devided by the sum of squared sample weights within
each cluster (see Carle (2009), Appendix B).
Carle AC. Fitting multilevel models in complex survey data with design weights: Recommendations. BMC Medical Research Methodology 2009, 9(49): 1-13
Aaparouhov T. General Multi-Level Modeling with Sampling Weights. Communications in Statistics<U+2014>Theory and Methods 2006, 35: 439<U+2013>460
# NOT RUN {
data(nhanes_sample)
scale_weights(nhanes_sample, SDMVSTRA, WTINT2YR)
# }
Run the code above in your browser using DataLab