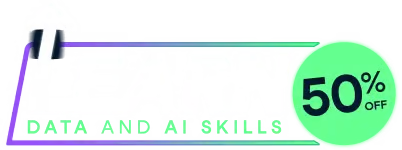
Last chance! 50% off unlimited learning
Sale ends in
shape1
and
shape2
(and optional non-centrality parameter ncp
).dbeta(x, shape1, shape2, ncp = 0, log = FALSE)
pbeta(q, shape1, shape2, ncp = 0, lower.tail = TRUE, log.p = FALSE)
qbeta(p, shape1, shape2, ncp = 0, lower.tail = TRUE, log.p = FALSE)
rbeta(n, shape1, shape2, ncp = 0)
length(n) > 1
, the length
is taken to be the number required.dbeta
gives the density, pbeta
the distribution
function, qbeta
the quantile function, and rbeta
generates random deviates. Invalid arguments will result in return value NaN
, with a warning. The length of the result is determined by n
for
rbeta
, and is the maximum of the lengths of the
numerical arguments for the other functions. The numerical arguments other than n
are recycled to the
length of the result. Only the first elements of the logical
arguments are used.shape1
shape2
[dpqr]beta()
functions are defined correspondingly. pbeta
is closely related to the incomplete beta function. As
defined by Abramowitz and Stegun 6.6.1
beta
). pbeta(x, a, b)
. The noncentral Beta distribution (with ncp
beta
for the Beta function.x <- seq(0, 1, length = 21)
dbeta(x, 1, 1)
pbeta(x, 1, 1)
## Visualization, including limit cases:
pl.beta <- function(a,b, asp = if(isLim) 1, ylim = if(isLim) c(0,1.1)) {
if(isLim <- a == 0 || b == 0 || a == Inf || b == Inf) {
eps <- 1e-10
x <- c(0, eps, (1:7)/16, 1/2+c(-eps,0,eps), (9:15)/16, 1-eps, 1)
} else {
x <- seq(0, 1, length = 1025)
}
fx <- cbind(dbeta(x, a,b), pbeta(x, a,b), qbeta(x, a,b))
f <- fx; f[fx == Inf] <- 1e100
matplot(x, f, ylab="", type="l", ylim=ylim, asp=asp,
main = sprintf("[dpq]beta(x, a=%g, b=%g)", a,b))
abline(0,1, col="gray", lty=3)
abline(h = 0:1, col="gray", lty=3)
legend("top", paste0(c("d","p","q"), "beta(x, a,b)"),
col=1:3, lty=1:3, bty = "n")
invisible(cbind(x, fx))
}
pl.beta(3,1)
pl.beta(2, 4)
pl.beta(3, 7)
pl.beta(3, 7, asp=1)
pl.beta(0, 0) ## point masses at {0, 1}
pl.beta(0, 2) ## point mass at 0 ; the same as
pl.beta(1, Inf)
pl.beta(Inf, 2) ## point mass at 1 ; the same as
pl.beta(3, 0)
pl.beta(Inf, Inf)# point mass at 1/2
Run the code above in your browser using DataLab