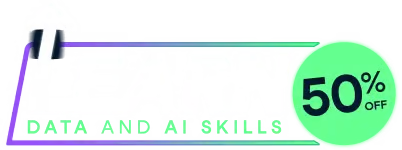
Last chance! 50% off unlimited learning
Sale ends in
A simple lookup table that stores embeddings of a fixed dictionary and size. This module is often used to store word embeddings and retrieve them using indices. The input to the module is a list of indices, and the output is the corresponding word embeddings.
nn_embedding(
num_embeddings,
embedding_dim,
padding_idx = NULL,
max_norm = NULL,
norm_type = 2,
scale_grad_by_freq = FALSE,
sparse = FALSE,
.weight = NULL
)
(int): size of the dictionary of embeddings
(int): the size of each embedding vector
(int, optional): If given, pads the output with the embedding vector at padding_idx
(initialized to zeros) whenever it encounters the index.
(float, optional): If given, each embedding vector with norm larger than max_norm
is renormalized to have norm max_norm
.
(float, optional): The p of the p-norm to compute for the max_norm
option. Default 2
.
(boolean, optional): If given, this will scale gradients by the inverse of frequency of
the words in the mini-batch. Default False
.
(bool, optional): If True
, gradient w.r.t. weight
matrix will be a sparse tensor.
(Tensor) embeddings weights (in case you want to set it manually)
See Notes for more details regarding sparse gradients.
weight (Tensor): the learnable weights of the module of shape (num_embeddings, embedding_dim)
initialized from
Input:
Output: *
is the input shape and
if (torch_is_installed()) {
# an Embedding module containing 10 tensors of size 3
embedding <- nn_embedding(10, 3)
# a batch of 2 samples of 4 indices each
input <- torch_tensor(rbind(c(1, 2, 4, 5), c(4, 3, 2, 9)), dtype = torch_long())
embedding(input)
# example with padding_idx
embedding <- nn_embedding(10, 3, padding_idx = 1)
input <- torch_tensor(matrix(c(1, 3, 1, 6), nrow = 1), dtype = torch_long())
embedding(input)
}
Run the code above in your browser using DataLab