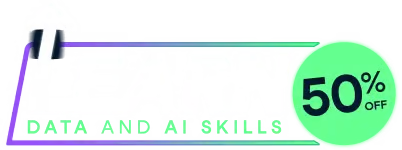
Last chance! 50% off unlimited learning
Sale ends in
decompTs(x, startyr, endyr, event = TRUE, type = c("mult", "add"))
A multiplicative decomposition will typically be useful for a biological community- or population-related variable (e.g., chlorophyll-a) that experiences exponential changes in time and is approximately lognormal, whereas an additive decomposition is more suitable for a normal variable. Aside from the long-term mean, each component of a multiplicative decomposition will average 1, whereas each component of an additive decomposition will average 0.
If event = TRUE
, the seasonal component represents a recurring monthly pattern and the events component a residual series. Otherwise, the seasonal component becomes the residual series. The latter is appropriate when seasonal patterns change systematically over time. You can use plotSeason
and seasonTrend
to investigate the way seasonality changes.
plotSeason
, seasonTrend
# Apply the function to a matrix time series,
# producing a list of decompositions
ans <- vector('list', ncol(sfbayChla))
names(ans) <- colnames(sfbayChla)
for(i in seq(along = names(ans))) {
ans[[i]] <- decompTs(sfbayChla[, i])
}
# A quick plot for a time series decomposiiton
plot(ans[[7]], nc = 1, main = paste(names(ans)[7], "Chl-a decomposition"))
Run the code above in your browser using DataLab