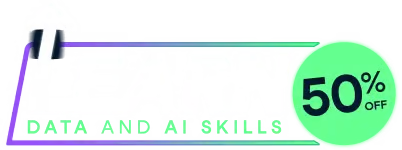
Last chance! 50% off unlimited learning
Sale ends in
pGPx
is a R package to generate pseudo-realizations of Gaussian
process excursions sets. The paper Azzimonti et
al. (2016) and the manuscript
Azzimonti
(2016)
provide explanations for the problem and the methods.
The package provides approximate posterior realizations over large designs by simulating the field at few well chosen points and interpolating. The simulation points are chosen minimizing the (posterior) expected distance in measure between the approximate excursion set and the full excursion set. The main functions in the package are:
optim_dist_measure
: computes the optimal simulation points e_1, … ,
e_m according to algorithm A or B.
krig_weight_GPsimu
: Given the simulations points and the
interpolation points computes the kriging weights for the approximate
process at the interpolation points.
grad_kweights
: Given the simulations points and the interpolation
points returns the gradient of kriging weights with respect to the
interpolation points.
expDistMeasure
: computes the expected distance in measure between
the excursion set of the approximated process and the true excursion
set.
simulate_and_interpolate
: Generates nsims approximate posterior
field realizations at the interpolation points given the optimized
simulation points.Contour length: the function compute_contourLength
computes the
excursion set contour length for each GP realization.
Distance transform: the function dtt_fast
computes the distance
transform of a binary image (Felzenszwalb and Huttenlocher, 2012) and
the function DTV
computes the distance transfom variability.
Volumes: the function computeVolumes
computes the excursion
volumes for each GP realization. It also applies a bias correction for
approximate realizations.
Azzimonti, D. and Bect, J. and Chevalier, C. and Ginsbourger, D. (2016). Quantifying Uncertainties on Excursion Sets Under a Gaussian Random Field Prior. SIAM/ASA Journal on Uncertainty Quantification, 4(1), 850-874. DOI: 10.1137/141000749. Preprint at arXiv:1501.03659
Azzimonti, D. (2016). Contributions to Bayesian set estimation relying on random field priors. PhD thesis, University of Bern. Available at link
Felzenszwalb, P. F. and Huttenlocher, D. P. (2012). Distance Transforms of Sampled Functions. Theory of Computing, 8(19):415-428. DOI: 10.4086/toc.2012.v008a019.
install.packages('pGPx')